Background
In March 2023, Gauntlet received a grant from the Uniswap Foundation to help research and recommend usage for a portion of the $20m grant budget allocated to help promote the growth of the Uniswap Protocol. As a first step of this grant, Gauntlet created and published a quantitative objective framework for the Uniswap Foundation and the broader community to use in deciding on and comparing potential proposals.
In this post, we provide a recommendation for how the Uniswap Foundation should use at least a portion of its allocated budget through the introduction of a liquidity incentives program which we will call “Liquidity Bootstrapping.” This recommendation aims to drive long-term growth at a positive ROI relative to token spend.
To generate this proposal Gauntlet performed 3 novel pieces of background research on the dynamics of on-chain liquidity providers and traders, which we used to decide our final recommendation.
Through this research, we were able to validate a series of assumptions to determine which types of programs made the most sense between direct trading incentives, direct liquidity provider incentives, or a hybrid approach incentivizing both Traders and LPs at the same time.
How to quantitatively select an incentives program?
In Gauntlet’s original objective function framework, we outlined the main users of the Uniswap Protocol as Traders and Liquidity Providers (LPs). First, we aim to decide which of these user types should be the focus of the growth strategy. We identified three potential design directions for incentive programs to pursue:
- Trade Mining - reward traders directly for volume
- Payment for Order Flow (PFOF) - reward routing networks for routing favorable traders
- Liquidity Mining - reward LPs directly for supplying liquidity to the protocol
The goal of any incentives program is not simply to generate a temporary boost for Uniswap but to generate long-term growth of the protocol. Thus, we identified two primary research questions which can help decide what type of incentives program makes the most sense:
- To what extent are traders in the DEX ecosystem elastic to price execution?
- To what extent are liquidity providers in the DEX ecosystem elastic to trading returns?
The rationale behind these questions is that all incentive programs achieve their effect by improving either price execution for traders or returns for LPs. The elasticity or “stickiness” that users exhibit with respect to these factors on the Uniswap Protocol dictates the long-term efficacy of the program. We aim to design a program that targets specific user types that will continue generating gains for the protocol after the program has ended. The remainder of this analysis will walk through each type of program and evaluate them in light of user elasticity data.
Trade Mining
One type of program that we have seen other protocols employ is direct trading incentives. Through these programs, a DEX may provide direct incentives to traders or fund development grants which improve the trading interface through a nicer user interface or developer experience.
The goal of this type of program would be to onboard new traders onto the Uniswap ecosystem through subsidized execution costs with the hope that they will stay and continue trading on Uniswap after the program has ended. This type of program has been employed on several smaller dexes such as JSwap and YuzuSwap but their small size and limited traction make them poor examples as a case study.
How did this fare for LooksRare?
A better example of this type of program working at a larger scale is LooksRare, which is a decentralized NFT exchange that in January 2022 launched a trading rewards program. LooksRare provided traders a claim on LOOKS tokens depending on their trading volume relative to market volume on a 24-hour basis. At the time, the program looked to be a strong success as it attracted many users to the LooksRare platform, which launched with a high degree of success based on its strong trading volume.
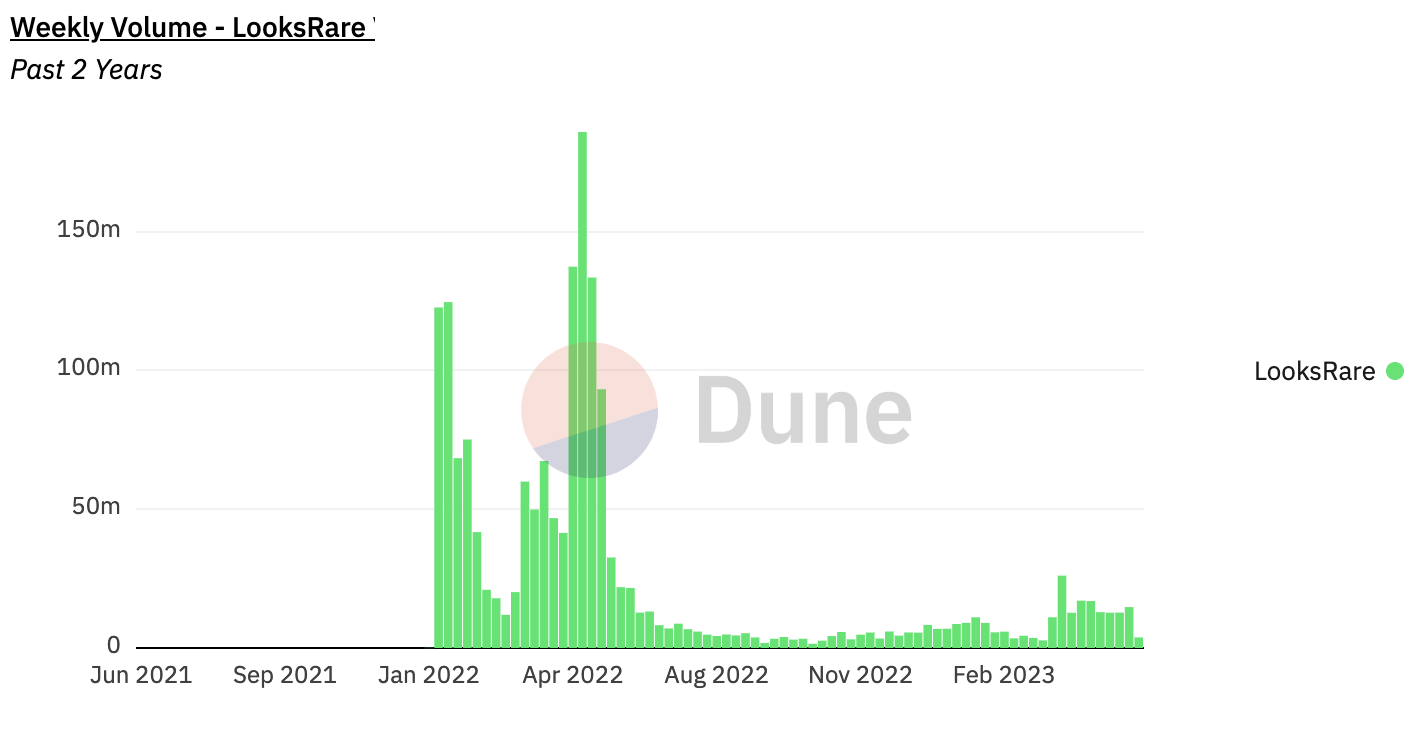
Source: https://dune.com/hildobby/LooksRare-VS-OpenSea
This program ultimately proved to be highly controversial however, as on-chain analysis concluded that due to the incentives program, a large amount of the trading volume on LooksRare turned out to be wash trading volume (NFTs traded between a single user in order to “farm” rewards, and not actual organic usage of the protocol).
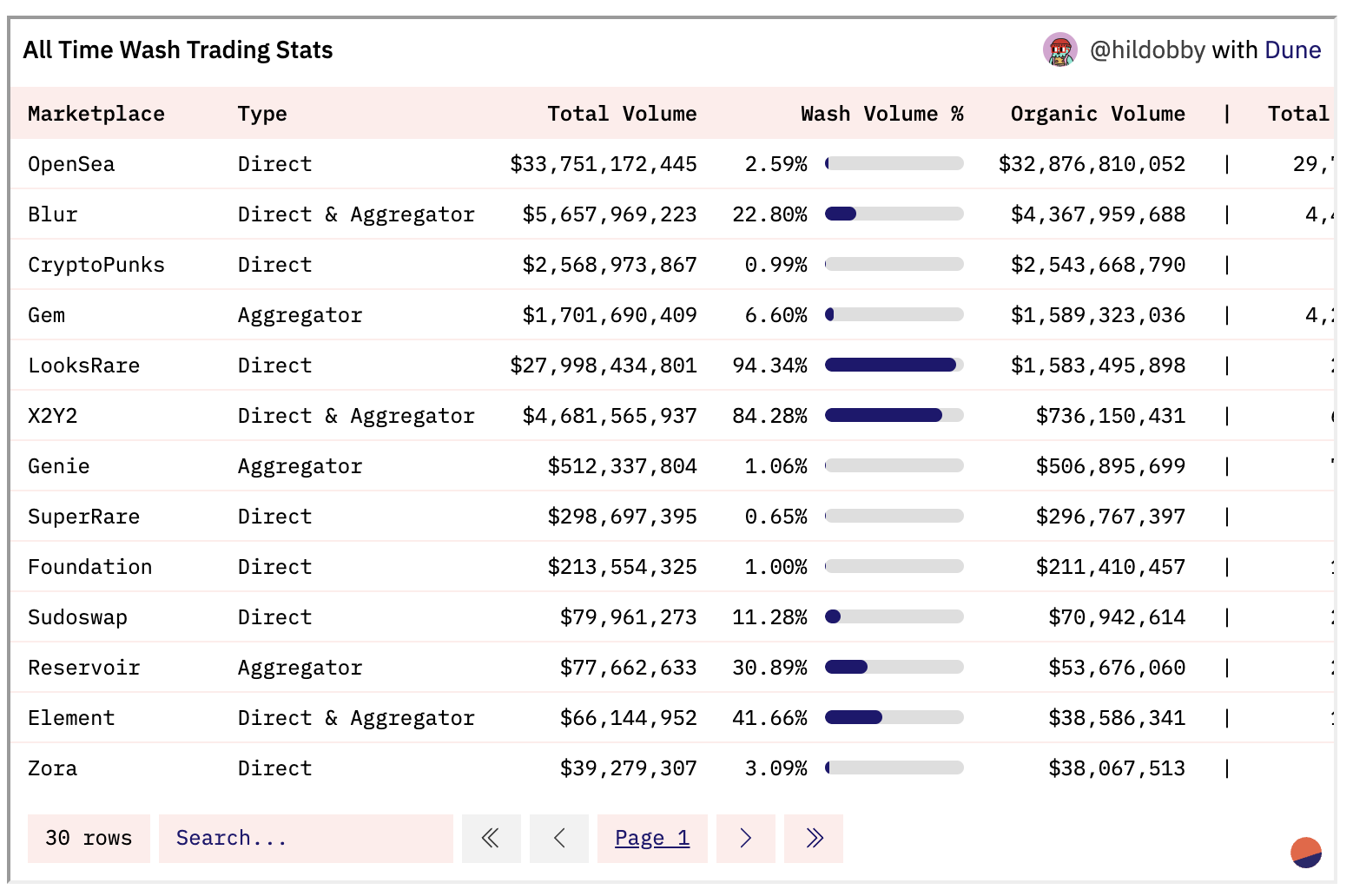
Source: https://community.dune.com/blog/nft-wash-trading-on-ethereum
This program ended in March of 2023 after significant pushback from the community around the wash trading taking place to earn these rewards and a severe reduction in all trading volume and the value of the LOOKS token.
What would a successful Trade Mining program require?
While trade mining has historically been unsuccessful at jumpstarting the usage of a protocol, there are Web2 examples of user-level usage incentives programs which are quite successful and ubiquitously acknowledged as having positive ROI. One can look at credit card points or coupons offered by ride-sharing apps like Uber or Lyft as successful examples of a company paying its users to use their product often taking an up-front loss. A successful “Trade Mining” program requires the two following statements to hold true:
- Sybil Resistance - The incentives program must be difficult or impossible to exploit
- Inelastic Users - Users tend to be “sticky” and continue using the product after incentives are removed
Sybil resistance requires good mechanism design and is likely achievable simply by ensuring that the cost of doing a trade is greater than the incentive for doing the trade. This type of program could be thought more of as a “subsidy” rather than an incentive since the returns for doing a trade are still nominally negative or close to zero. Sybil-resistant mechanisms that provide excess incentives are difficult to implement due to the anonymous nature of crypto but may be possible through some complicated wallet tagging schemes. However, such programs are usually not 100% effective at deterring adversarial users, as we can see from the case of LooksRare as well as the difficulties experienced both by Uber (see fake users gaming the system) and credit card companies alike (see credit card churning).
In addition to the difficulty of designing a Sybil-resistant system, this type of program would also require the users (traders in Uniswap’s case) to be “sticky” in that they will continue to prefer to use Uniswap after incentives are shut off. If this were the case, we would expect that there exist traders in the DeFi space who are currently routing their orders “inaccurately” in that they are making a swap at an exchange that results in sub-optimal price execution, likely as a result of some “brand” effect of preferring to use the exchange they had previously onboarded to.
Due to the challenges laid out above, it would be difficult for Uniswap to create an effective trade mining program that is sybil resistant and targets inelastic users. To make the program sybil resistant, the following options could be considered:
- Identity Verification: Users would be required to undergo a thorough identity verification process, linking their accounts to verified identities, making it difficult to create multiple accounts and exploit the program. However, requiring identity verification compromises user privacy and goes against the decentralized nature of blockchain protocols, potentially deterring users who value anonymity and imposing legal and compliance challenges.
- Proof of Stake: Users would need to lock up a certain amount of tokens as collateral to participate in trade rewards, increasing the cost for adversaries to create multiple accounts. However, implementing a PoS mechanism introduces barriers to entry, favors users with larger token holdings, and may discourage smaller traders, limiting program effectiveness, while determined adversaries with substantial holdings may still find it economically viable to exploit the program.
- Activity Patterns Analysis: Trading patterns and other behavioral data would be analyzed to identify suspicious accounts engaged in exploitative behaviors using statistical models. However, building a model that could reliably identify adversaries is challenging when malicious users can continuously adapt their behavior to mimic normal users.
To make the program encourage user stickiness, the following options could be considered:
- Novice User Targeting: Uniswap could advertise this program to retail traders who are new to DeFi, who may be less likely to seek out DEX alternatives to Uniswap after the incentive period is over. However, user stickiness is not guaranteed through this method, and it is unlikely that these novice users would provide significant trading volume relative to more experienced traders.
- Loyalty Rewards Program: Uniswap could introduce a loyalty rewards program where users earn additional benefits or privileges (such as lower trading fees) based on their trading volume or length of engagement with the platform, incentivizing them to continue trading on Uniswap even after the trade mining program ends. However, while a loyalty rewards program may encourage user stickiness, it may also create an expectation of ongoing incentives, and once these rewards are removed, users may be less inclined to continue trading exclusively on Uniswap, especially if competing platforms offer more attractive features or lower fees.
- Community Engagement: Uniswap and the Uniswap Foundation have historically engaged with users through forums, events, and governance mechanisms. Uniswap could further invest in these efforts, which could cause users to feel a deeper connection with Uniswap and thus develop stronger brand loyalty. However, cost savings and financial incentives offered by competing platforms likely outweighs any achievable sense of community ownership, potentially leading them to explore alternative options.
Considering the challenges involved in designing a sybil-resistant system and the uncertainty surrounding user stickiness, it becomes increasingly difficult to envision a trade mining program for Uniswap that would achieve lasting success beyond the subsidy period.
Measuring Swapper Price Elasticity
In order to determine the efficacy of a Trade Mining program, Gauntlet assessed the elasticity or “stickiness” of traders relative to their price execution. If traders tend to perform swaps outside of Uniswap that could have received a better price on Uniswap, this would imply the existence of “sticky” traders who may be induced to enter the Uniswap ecosystem through Trade Mining rewards.
To assess the price efficiency of DeFi swappers, Gauntlet carried out an analysis of 1.9 million swaps spanning four decentralized exchanges (dexes) - Uniswap v2/v3, Sushiswap, Balancer, and Curve. This analysis covered ten major trading pairs, including ‘STETH_WETH’, ‘OHM_WETH’, ‘SYN_WETH’, ‘DAI_USDC’, ‘DAI_USDT’, ‘WBTC_WETH’, ‘USDT_WBTC’, ‘FRAX_USDC’, ‘USDC_USDT’, and ‘USDT_WETH’. These pairs represent a trading volume of $69.473 billion from September 14, 2022, to February 27, 2023.
Harnessing the Gauntlet Simulation Platform, we reran the 1.9 million swaps on a simulated version of Uniswap v3, using detailed liquidity data from the exact minute each swap originally occurred. To ensure the accuracy of our simulation, we tested the calibration by re-routing the real swaps through the simulated v3. In an ideally calibrated simulation, we’d anticipate no difference (0% error) between the actual and simulated outcomes of a swap.
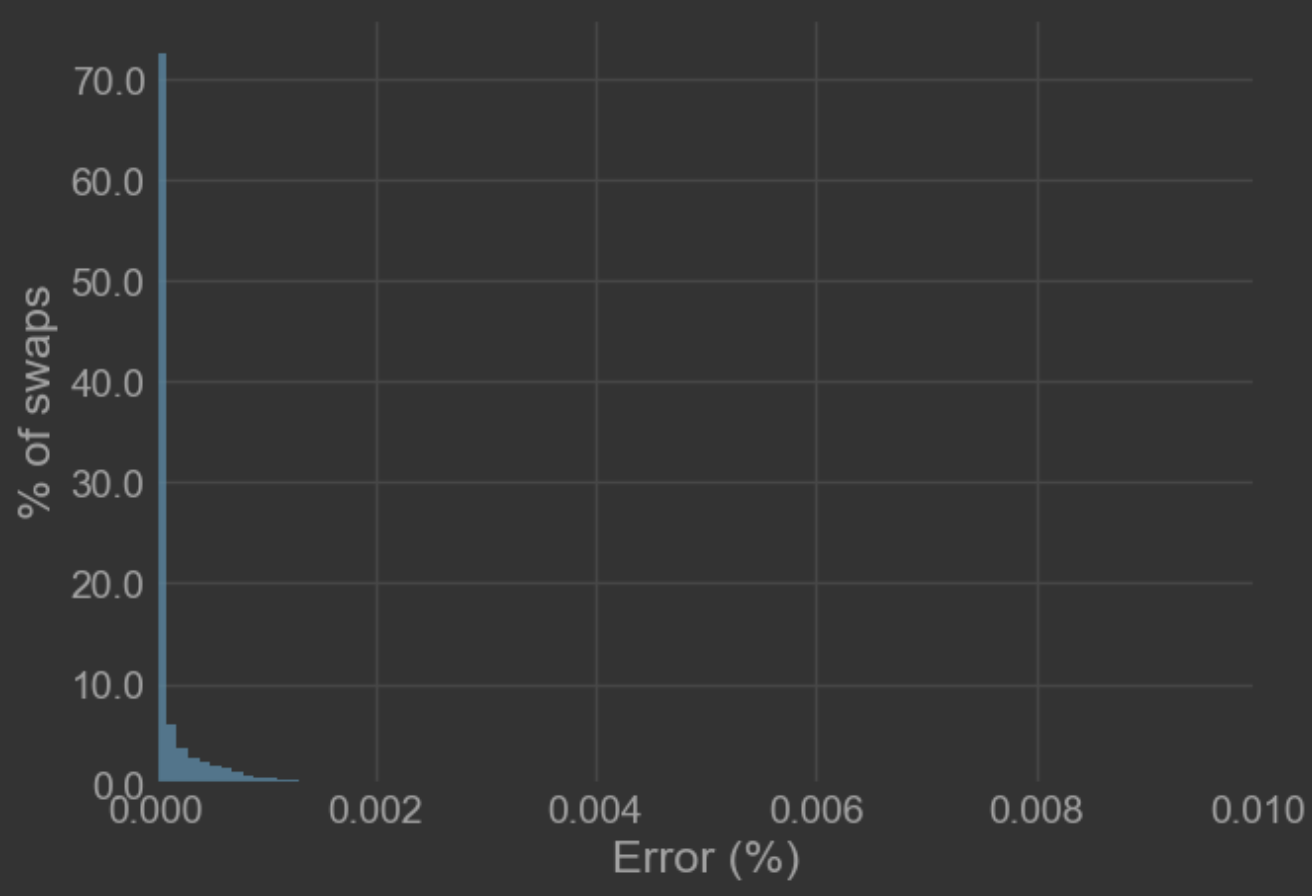
Our simulation proved to be well-calibrated, with less than 10 basis points (bps) of error in 99% of swaps and less than 1 bp of error in 90% of swaps. The observed error likely stemmed from complex swaps involving sandwich attacks or other miner extractable value (MEV) issues that impact the execution price due to transaction order within the block. We used Nansen’s MEV tagging to filter out MEV tagged swaps in this calibration step.
Applying this method, we then determined the percentage difference between trades executed on rival exchanges (Curve, Balancer, and Sushiswap) and the predicted outcomes from our simulation had the volume been routed through corresponding Uniswap v3 pools.
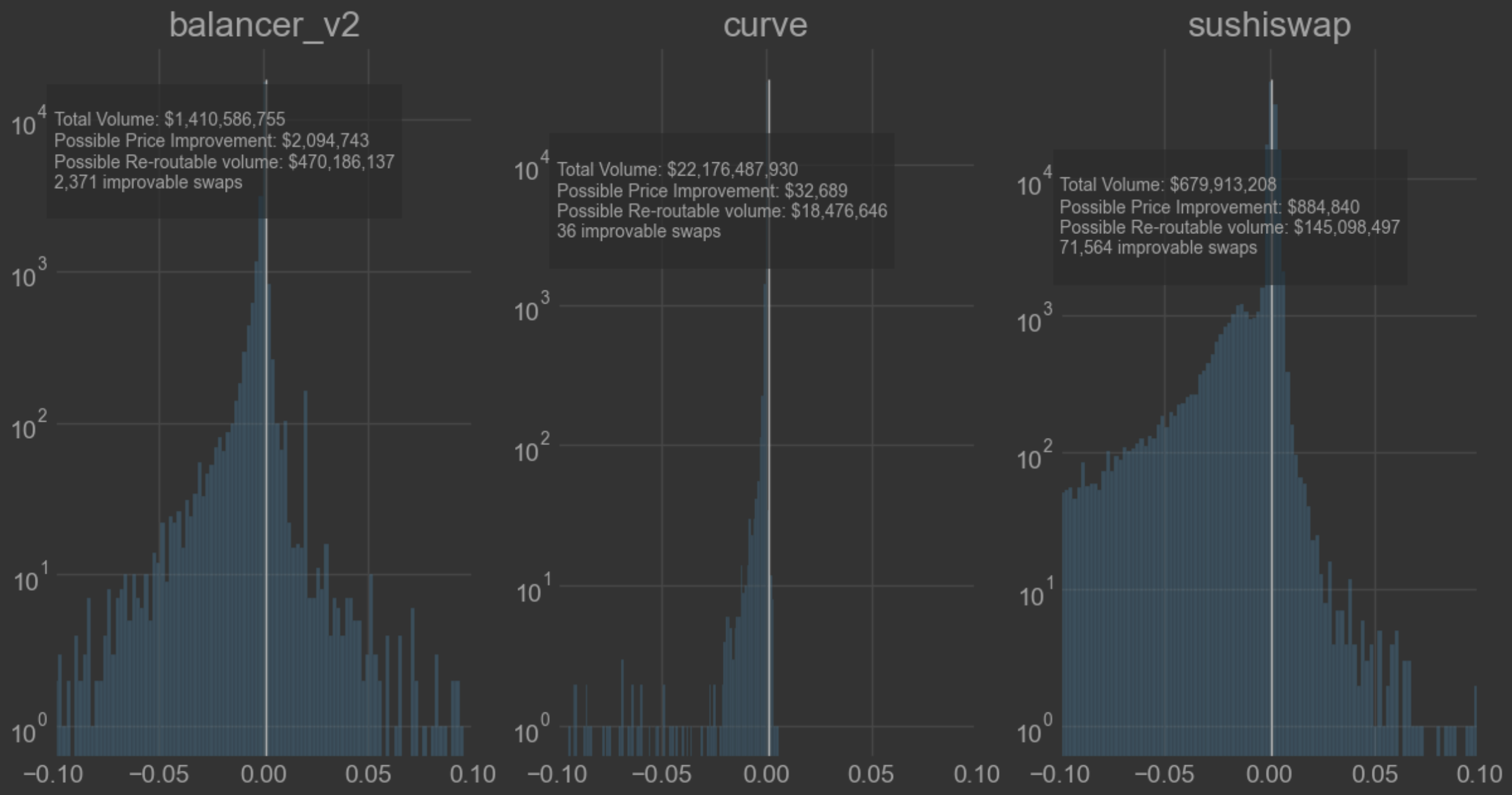
We identified several swaps on competing exchanges that were executed outside our 10bps error margin, totaling $633m in trading volume that seemed to be sub-optimally routed. Overall this represents about 1% of the total DEX trading volume on ETH mainnet, which is not significant in our analysis.
The lack of inefficient volume is compounded when we examine the types of users trading on these exchanges. To do so, we grouped users into cohorts first by tagging MEV originating swaps as MEV, then bucketing wallets by overall trading volume across our 6-month dataset as Whale (>$1m), Heavy (>$1k), and Retail (<$1k).

Despite whale or MEV-tagged addresses making up only 8% of users, about 94% of the volume originates from these addresses. To eliminate the possibility of incorrectly tagged MEV addresses, we also performed the same analysis with MEV removed as a possible cohort and found similar results. Whale traders represented only 1% of overall users but 91% of the overall trading volume.

This finding raises concerns about the presence of “sticky” traders. With most Mainnet swap volume originating from large whales (> $1m over six months) or MEV bots, it’s improbable that these groups would consistently use Uniswap due to a one-off onboarding incentive program. However, it’s worth noting that order routing protocols like 1Inch and Matcha, which route all orders from the same address, would fall into the whale category.
We revisited our initial analysis of reroutable volume by exchange, adding the user cohort dimension. This helps us identify where this reroutable volume originates, using 10bps as our significant marker based on our earlier established error threshold.

Our analysis shows that 92% of the reroutable volume originates from MEV or Whale traders, with only ~$53m of potentially misrouted volume originating from retail or heavy traders. MEV or Whale traders in general are unlikely to show high loyalty to specific exchanges leaving us with a very small amount of volume that could be enticed to move to Uniswap and remain sticky.
This leaves us with only $53m in inefficiently routed volume from retail or heavy dex traders, which Uniswap could likely attract long term through some trader-mining rewards program, only 0.07% of the overall dex volume we observed. Traders on ETH mainnet seem to be very efficient at routing their orders for best execution and not “sticky” in any material way that we could observe.
Does trade mining make sense on Uniswap?
Our analysis strongly suggests that running a trade mining or trader onboarding program for Uniswap currently isn’t warranted. We found no significant volume from users likely to be “sticky” on alternative exchanges that Uniswap could attract through such a program.
It is possible that there are traders who exist outside of the DeFi DEX ecosystem who may be induced to join as a result of trading mining incentives, but absent evidence that this cohort of traders exists and given the past failures of trade mining programs, it is unlikely that a trade mining program will be effective for Uniswap.
Payment for Order Flow
Another option we evaluated was incentive spending via paying aggregators such as 1Inch or Matcha to preferentially route orders to Uniswap, using a mechanism common in the traditional finance world known as “Payment for Order Flow”.
What is Payment for Order Flow?
Payment for Order Flow (PFOF) is a practice in the financial industry where brokerage firms receive compensation for routing their clients’ orders directly to market makers for execution. Essentially, it is a fee paid by market makers for the right to execute client orders from specific brokerage platforms.
A market maker who engages in PFOF does not earn money by providing bad price execution, as this is not long-term sustainable after traders wisen to the game. Instead, market makers engage in PFOF in order to avoid executing unfavorable trades, which are statistically less likely to originate from certain venues. To see how this works, consider two identical-looking orders from two different traders:
Order 1
Size: $100,000
Side: Sell
Asset: TSLA
Trader: Elon Musk
Order 2
Size: $100,000
Side: Sell
Asset: TSLA
Trader: A dentist who didn’t like one of Elon Musk’s recent tweets
While the orders may appear identical (a $100k sell order of TSLA), the market maker should be much more fearful of executing Elon Musk’s order than the dentist’s order.
A sell order from an insider like Elon Musk is much more likely to be important to the future price of TSLA. For example, this may be only the first of many sell orders that will move the price lower. In some cases, an insider sell order may itself be impactful, as other traders closely watch reported holdings and trades. This increases the risk that the price will drop before the market maker can liquidate their new inventory, so buying TSLA stock from Elon Musk is a risky proposition.
The dentist’s order, on the other hand, likely does not represent any important information and is essentially random. There is no particular risk of the price dropping in reaction to the dentist’s order and thus less risk in trading with the dentist than with Elon Musk. Formally, this phenomenon is known as “Order Toxicity”, which represents the increased risk of trading with highly informed counterparties versus random flow.
In traditional finance, orders on public exchanges are matched anonymously, so it is impossible for a market maker on a public exchange to differentiate between informed insiders like Elon Musk and uninformed traders like day-trading dentists. To execute more trades, some market makers will pay brokerages known for having uninformed traders to route orders directly to the market maker. The market maker can then provide price execution equal to or better than the public market, since order flow routed through PFOF is materially less risky to trade against.
PFOF is profitable for market makers because it allows them to reduce the risks related to highly-informed traders. The market maker can earn a higher expected value from executing trades through PFOF even after accounting for price execution and the fees paid to the broker. The ability to differentiate between informed and uninformed traders is the core reason PFOF is practiced in traditional finance.
Payment for Order flow on Uniswap
On Uniswap, the exchange mechanisms are different – instead of market makers, Uniswap has Liquidity Providers (LPs). However, these LPs face similar risks as traditional market makers concerning the toxicity of flow. Highly-informed traders who trade against the liquidity on Uniswap are more likely to generate losses for the LPs. In this context, informed traders can be anything from market insiders to arbitragers taking advantage of price discrepancies between exchanges. These trades on Uniswap are generally unprofitable to LPs and reflect a risk that must be compensated by non-toxic flow from “random” traders.
In general, increasing the share of non-toxic flow to liquidity pools on Uniswap would benefit LPs by providing a source of fee revenue that is not associated with increased risk.
What would PFOF look like on Uniswap?
Max Holloway, on behalf of Xenophon Labs, wrote a paper quantifying the value that LPs get from additions to non-toxic flow (Section 2 Nontoxic Orderflow’s Value to LPs), various methods to increase non-toxic flow through developer mining (Section 4.1/2/3 How to Incentivize Nontoxic Orderflow), ultimately suggesting that a developer mining program doesn’t make sense (Section 3.1-3 Nontoxic Orderflow’s Value to the Protocol and Sections 4.1-5 Recommendation) until the Uniswap fee switch is turned on.
As Max points out, paying to increase non-toxic flow on Uniswap would increase expected LP returns and likely increase available liquidity on the Uniswap Platform. If we assume that LPs are elastic to changes in returns, this would drive increased amounts of liquidity to the Uniswap DEX. Increased liquidity begets more volume, increasing potential future revenue or governance for the Uniswap protocol and UNI token. This provides a quantitatively calculable benefit to the Uniswap protocol that can be derived from PFOF.
An additional benefit may exist in incentivizing additional integrations from routing protocols. In a world where traders are currently not getting the best price execution, paying some platforms to integrate with Uniswap may induce them to begin correctly routing their orders. This one-time cost to induce more integrations may lead to the long-term benefit of Uniswap by reducing the existence of orders poorly routed to alternative venues. Given our evidence of highly efficient traders discussed earlier this benefit is unlikely to move the needle long term as traders ultimately route their volume efficiently rather than to the venue that they currently have an integration with.
Payment for Order Flow vs. Liquidity Mining on Uniswap?
If the primary goal of PFOF on Uniswap is to use re-routed volume to incentivize more liquidity, we can also consider a more direct way to incentivize liquidity – Liquidity Mining Incentives. With Liquidity Mining, Uniswap could directly distribute UNI tokens to LPs to subsidize the risk they take by providing liquidity. The pros and cons of Liquidity Mining versus PFOF include several dimensions:
Efficiency
Using PFOF, there are several intermediaries that must be paid before benefit ultimately accrues to LPs. The interface which is re-routing the order to Uniswap must be paid to set up integrations, as well as on a per-trade basis. The trader whose trade is re-routed must either receive price improvement or be compensated with some form of incentive for using the program.
The LPs can also achieve higher returns from the reduced risk of toxic order flow and increased fees. However, they will likely not receive 100% of the possible benefit since there will still be some toxic flow that occurs through PFOF.
It is unlikely that $1 of incentives spent on re-routing orders towards Uniswap will accrue at least $1 in value to LPs unless a significant portion of orders are currently poorly routed. This is due to the fact that any order which is re-routed by a PFOF program must receive price improvement or incentives to make it economically viable. Unless a significant amount of mis-routed flow exists, this will be reflected as lower efficiency in incentivizing liquidity through PFOF versus through direct liquidity mining.
Targeting
With PFOF, liquidity is incentivized through increased and less-toxic order flow. However, this benefit is spread broadly across all the resulting trades and cannot be targeted to any specific type of LP. Because of this, we would expect certain LPs to benefit from PFOF more than others. Sophisticated LPs capable of providing JIT liquidity or actively managed price ranges will likely be able to capture more trades and more value from PFOF programs.
This is not necessarily a bad thing, but makes it difficult to target incentives to where they produce the maximal impact. It is likely that certain characteristics of LPs give more value to the protocol, such as passively providing liquidity versus actively.
With a direct liquidity mining program, Uniswap can pick and choose exactly where and how incentives are spent, directing incentives only to LPs that it thinks are most long-term beneficial for the protocol. With PFOF, this targeting does not exist, as all liquidity can be used to execute any given order.
Long Term Integrations
In a world where aggregators like 1Inch or centralized brokers are routing orders poorly and could obtain price improvement on Uniswap, paying these parties to rectify the situation could make sense. The startup cost of setting up a Uniswap integration may be high relative to the improvements in price execution, and so using PFOF as an incentive could be a reasonable way to overcome this burden.
This benefit relies heavily on the assumption that trades are currently being routed poorly. If trades are currently being routed optimally, paying for integrations is unlikely to move the needle since the integrations either already exist or are not economically viable in the first place.
Even assuming trades are being routed poorly, it is questionable that PFOF is the most cost-effective way to rectify this issue. Alternatively, a one-off incentive to aggregators may also work to cover the integration cost without incurring the longer-term costs of a PFOF program.
Given our earlier conclusion that trades seem to be routed fairly efficiently on Mainnet, there is little evidence to support a substantial benefit from PFOF through this mechanism.
Does PFOF make sense on Uniswap?
PFOF on Uniswap has potential benefits, such as incentivizing additional integrations from brokers and increasing LP returns, which could lead to increased liquidity and volume on the platform long term. However, it also comes with several drawbacks, such as reduced efficiency in incentivizing LPs and a lack of targeting ability to direct incentives to specific LPs with dubious returns to creating new integrations based on our finding that DEX volume is already routed accurately.
Liquidity Mining
Gauntlet sees liquidity mining as the more promising approach for driving Uniswap’s growth moving forward. Liquidity Mining (LM) is an incentive strategy in which rewards are paid out to liquidity providers. Typically, liquidity mining involves setting a fixed budget of tokens to be distributed to LPs proportional to the amount of liquidity they staked in the protocol over a set period of time.
How can Liquidity Mining drive long-term value?
There are 2 mechanisms Gauntlet has identified which would result in this long-term sustained growth due to a liquidity mining program.
Forgetful Liquidity Providers
One possible theory by which you would achieve a sustained lift from a liquidity mining program is through Forgetful LPs, whereby LPs transfer liquidity to pools in order to capture incentives and subsequently “forget” that their liquidity exists and fail to rebalance their portfolio after the incentives program ends.
This may be observable in some long-lasting liquidity mining programs but is unlikely to be observed in such a short-term (several weeks) program where the end date of the program was known from the start.
Liquidity Bootstrapping
Liquidity mining incentives can be useful in bootstrapping liquidity that sticks around after incentives are removed when the temporary boost in liquidity incentivizes more volume. Specifically, we hypothesize that the following chain of events can occur:
- Liquidity mining incentives introduced
- LPs add liquidity to the pool, which improves execution quality for traders
- Traders route more of their swaps through the pool and thus pay more fees to LPs
- Fees from traders further incentivizes liquidity in the pool until a stable equilibrium between liquidity and returns (fees + incentives) is reached.
- Liquidity mining incentives are removed, resulting in some liquidity being removed due to the lost incentives. * However, the new equilibrium between liquidity and fees is higher than before since the new fees keep some new liquidity in the pool, and that new liquidity keeps the trading volume flowing.
If this theory were true, we would expect to see increases in liquidity as well as trading volume (and by proxy trading fees) in pools where liquidity mining programs were run during the incentives period and a subsequent decrease in liquidity and trading volume after the incentives are removed (however a higher liquidity/fee equilibrium than the pool experienced before the incentives period). This theory requires 2 things to hold true:
- Liquidity must increase as a result of liquidity mining incentives
- Trading Volume (fees) must increase as a result of the increase in liquidity.
Liquidity Mining on Optimism Case Study
In late 2022 and again in early 2023, Uniswap conducted a liquidity mining experiment on Optimism in which OP incentives were distributed to LPs of specific pools through four liquidity management protocols. The results of the experiment were mixed: it was clearly observed that liquidity in all relevant pools appeared to increase throughout the duration of the incentives period and subsequently decrease after the incentives period was concluded. Prior analysis by community members focused on the trend of TVL significantly decreasing for most pools after the incentives period finished, concluding from this that the incentives program had been ineffective at creating sticky liquidity or any long-term flywheel effect.
From prior analysis, it would be natural to conclude that a liquidity mining rewards program is ineffective at generating a long-term “flywheel” of sustained lift in liquidity and volume for their protocol. However, Gauntlet re-examined the data for the Optimism liquidity mining experiment and arrived at a different conclusion for certain pools.
Our full analysis is posted here and summarized below.
Approach
We identified and attempted to correct 2 primary flaws in the existing research:
- The existing research did not account for or normalize overall market trends for the pairs relevant to the experiment
- The existing research did not analyze the dynamics of trading volume in relation to improvements in liquidity
In order to improve on the previous analysis and account for the noise of market fluctuations affecting liquidity and trading volume, we paired each pool that was part of the incentive program with a control pool that shares similar market characteristics which was not part of the incentive program. We can view the pools with incentives as being the “treatment group,” while pools without incentives were the “control group.” These pairs are shown below.
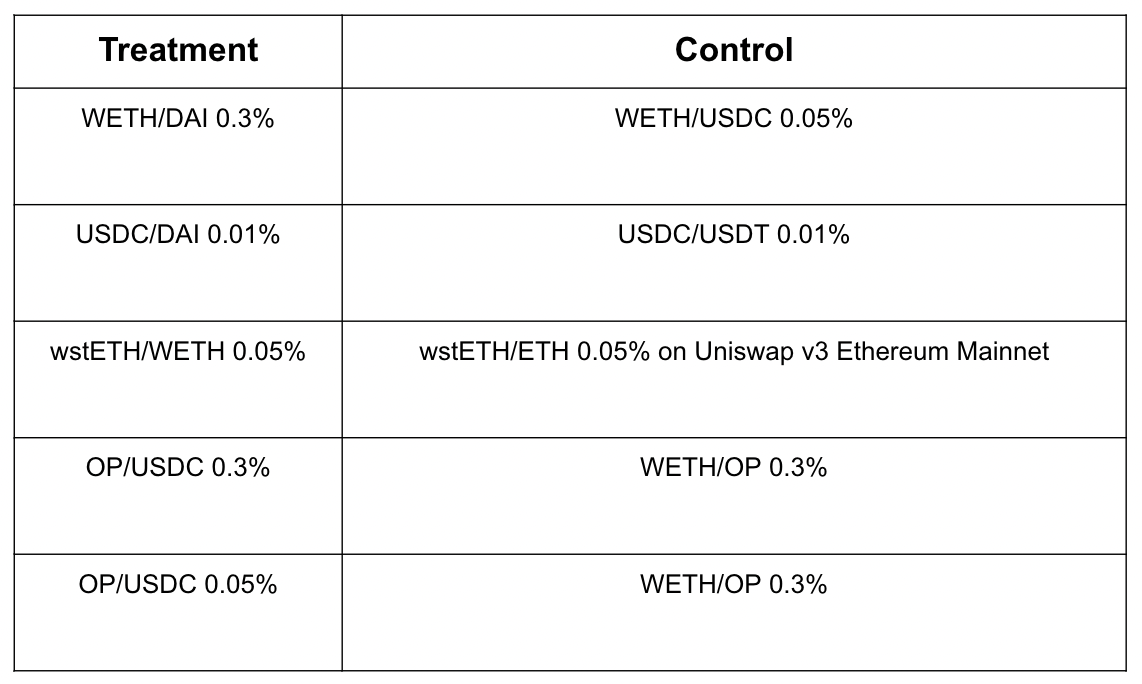
We computed a time series of each treatment pool’s relative share of TVL and volume given its control pool, which accounts for deviations in usage that affect the tokens at hand or Uniswap as a whole. We then performed a one-sided t-test to see if there was a meaningful increase in volume or TVL after the incentives started or if there was a meaningful increase in volume or TVL after the incentives ended (relative to levels before the incentives started). If liquidity mining was successful, then we expect to see that TVL increased during the experiment, leading to trading volume increasing during the experiment, leading to TVL and trading volume increasing after the experiment relative to before the experiment.
Results
Out of the 5 liquidity pools in the experiment, 2 of them (wstETH/WETH .05% and OP/USDC 0.3%) experienced a statistically significant sustained lift in both TVL and volume market share as compared to economically similar pools.
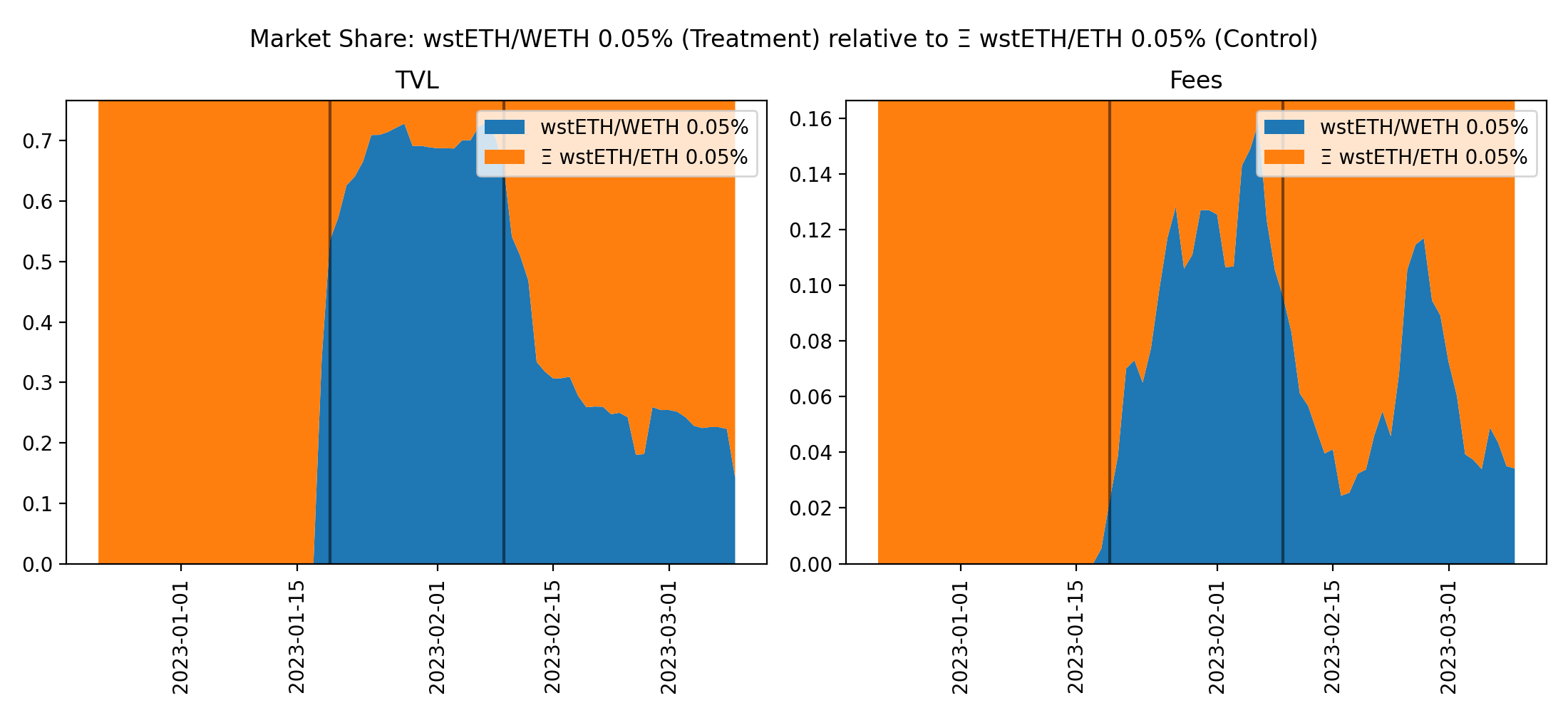
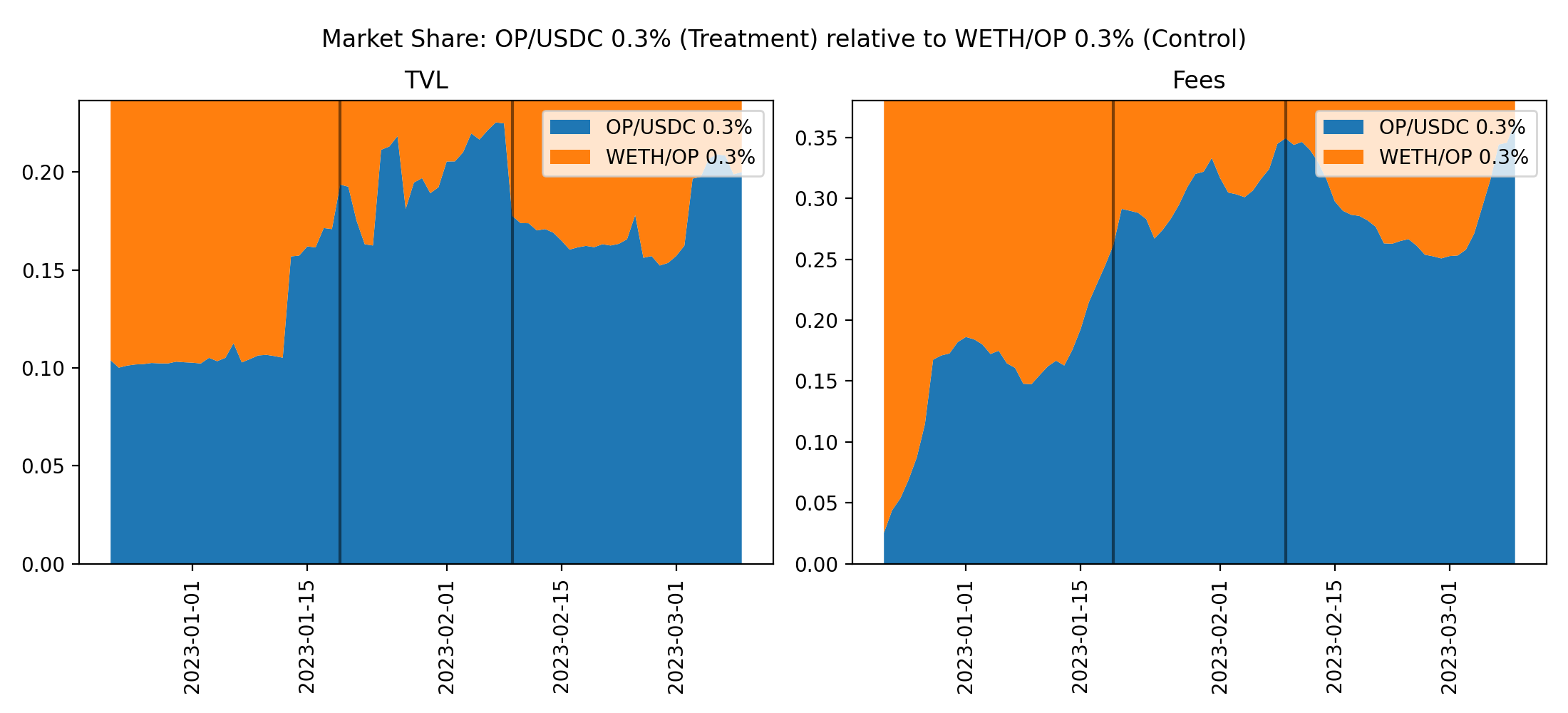
Three pools did not experience sustained lift (USDC/DAI 0.05%, WETH/DAI 0.05%, and OP/USDC 0.01%) for reasons that cannot be fully explained by our analysis. It is possible that one of several possible factors played a role either in confounding our analysis or preventing the effect from taking root. Some possible culprits may be a poor control pool analog, external incentives programs, cannibalization of liquidity or volume due to the incentives program, a lack of natural trading volume to route to these pools, or some other exogenous cause not included in this analysis. Future analysis efforts will be necessary to conclusively decide whether liquidity mining was a success or failure for these pools.
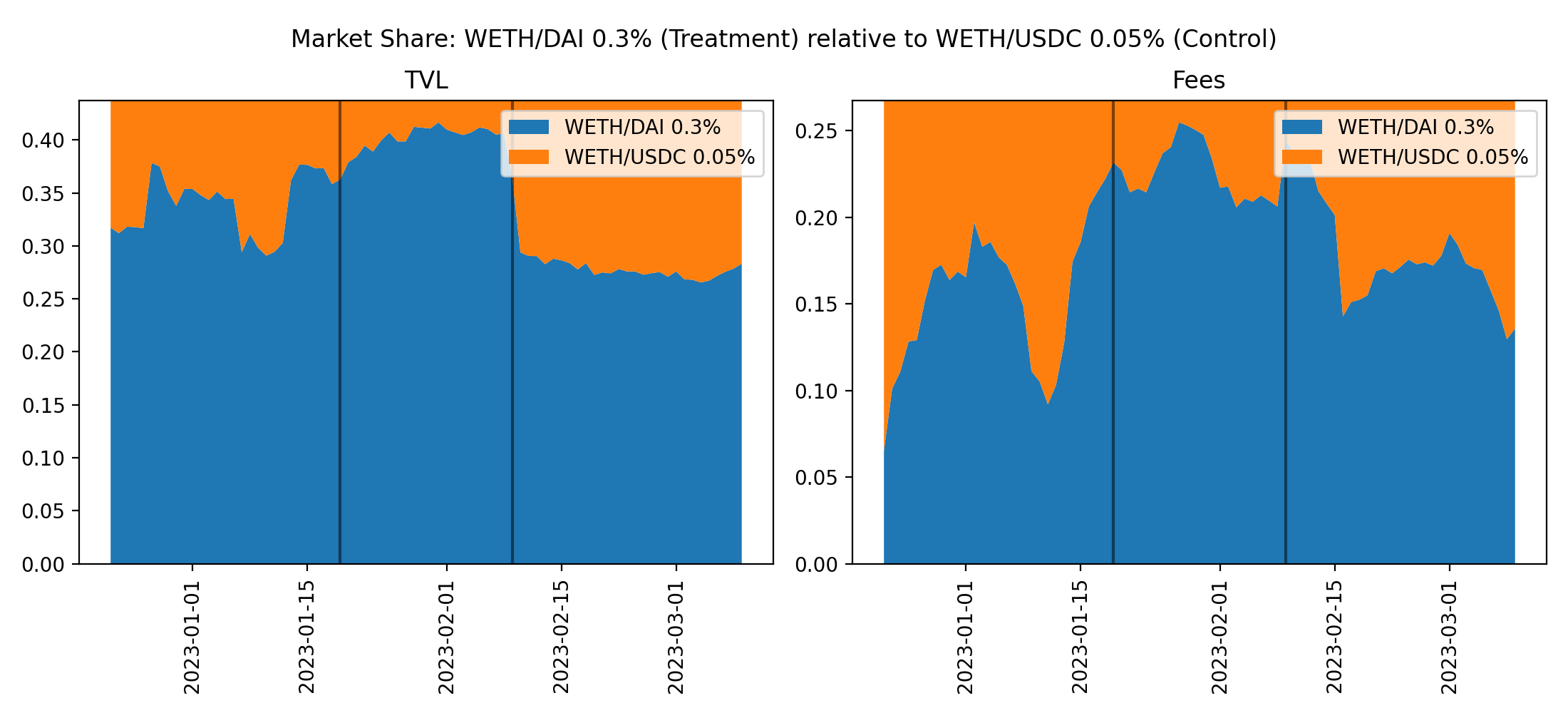
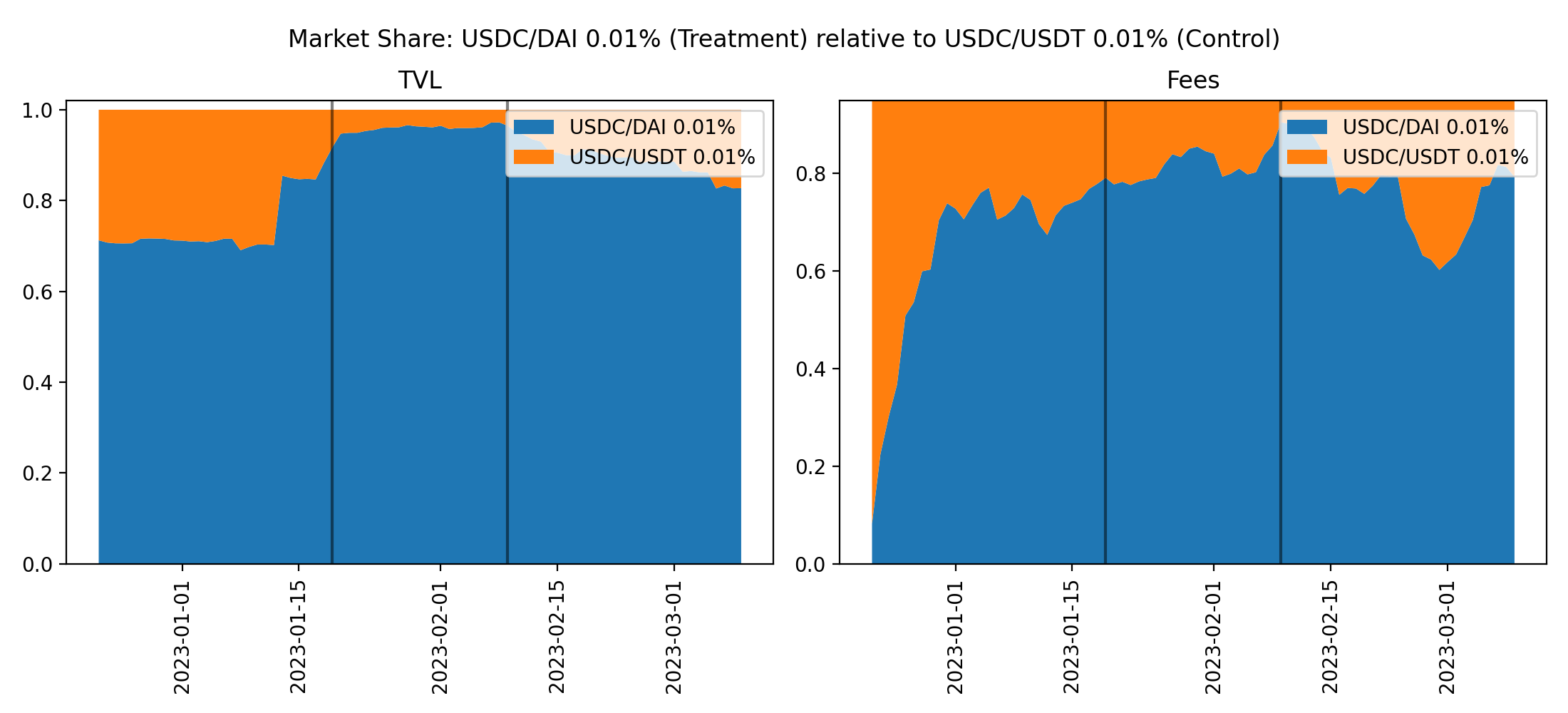

It is encouraging, however, that in this somewhat randomly selected experiment, we had a 40% success rate out of 5 pools in generating sustained impact through a liquidity mining incentives program. While a study of 5 pools cannot be conclusive, it supports the case that liquidity mining can be effective when pools are optimally selected through relevant analysis. We can the consider how to pre-identify pools that may be good candidates for liquidity mining and how to approach incentivizing them.
Pool Selection Methodology
The goal of liquidity mining is to maximize the Uniswap objective function as defined in our forum post. To do so, we need to maximize the gain in discounted protocol fee revenue (assuming the fee switch is on) while minimizing the spend of UNI tokens. This optimization needs to be done across the following parameters:
- Which pools do we incentivize?
- How much do we incentivize each pool?
- How long do we run the experiment for?
Our Approach
In order to bring in additional fees for the protocol, we need to increase Uniswap’s future trading volume. Additional trading volume can come from the following sources:
- Competing DEXes (Curve, Sushi, Balancer, etc)
- Competing CEXes
- Uniswap V2 and V1 pools
- New trading demand
Of these sources, competing DEXes provides the greatest opportunity: our trader elasticity analysis showed that on-chain traders tend to be efficient, so by improving trade execution quality, we can increase volume. Execution quality would be improved with more liquidity, and providing liquidity mining incentives, we can provide higher yield to LPs which would increase Uniswap’s liquidity.
The goal of candidate pool selection is to find ways to redirect trading volume from competing DEXes to Uniswap. Therefore, we are targeting Uniswap pools corresponding to pairs that have a high market-wide trading volume, but a low Uniswap v3 marketshare of trading volume.
Simulation
To help us evaluate the choice of incentive spend, we built a simulation that predicts the net benefit of incentivizing pool P given a daily spend amount S. The simulation works as follows:
- Initialization: for P and all competing DEXes that involve the same trading pair, get current liquidity (TVL) and volume (average daily volume, or ADV)
- Simulate the N days of the incentive period, doing the following for each day:
- Use the Liquidity Model to update the TVL of P given the pool ADV and incentive spend S
- Use the Volume Model to update the ADV of P given the pool TVL
- Simulate M days of the pool after the incentive period ends, doing the following for each day:
- Use the Liquidity Model to update the TVL of P given the pool ADV and 0 incentive spend
- Use the Volume Model to update the ADV of P given the pool TVL
- Compute the net benefit to the protocol via the Uniswap objective function using the timeseries of simulated ADV
Liquidity Model
The Liquidity Model determines pool TVL given ADV and incentive spend, and it works by assuming perfect elasticity for LPs, meaning that LPs would increase liquidity in a pool in order to maintain the same level of yield that is currently being earned. Yield is the sum of token incentives and trading fees. We assume that LPs use a rolling window of 14 days to compute trading fees, as this was the average update time we saw for whale LPs of major pools from our Mainnet LP Timing Analysis. After the first phase of the liquidity mining incentive is complete, we plan on building a more sophisticated Liquidity Model by fitting a statistical model to the data we collect on LP positions over the course of the experiment.
Volume Model
The Volume Model determines pool ADV given TVL, and it assumes that a 1% increase in liquidity market share leads to a 1% increase in volume marketshare. The market share for a pool is the percentage of liquidity or volume that the pool gets against all other pools that involve the same trading pair across all competing DEXes on the same chain. This linear relationship between the market shares of liquidity and volume is validated by the results of our Optimism LM analysis. In the future, we plan on building a more sophisticated Volume Model that reroutes historical swaps to the DEX that provides the best execution quality given its liquidity.
Visualization
The figure below demonstrates a successful liquidity mining scenario according to our simulation. Specifically, this simulation run corresponds to the DAI/USDT 0.01% pool given an incentive spend of $6314. The top figures show raw ADV and TVL, while the bottom figures show the pool’s market share of ADV and TVL. The x-axis is time in days: t=0 corresponds to the period before incentives are introduced, t=1 corresponds to the first day of incentives, and the black dotted line shows the last day of the incentive period.
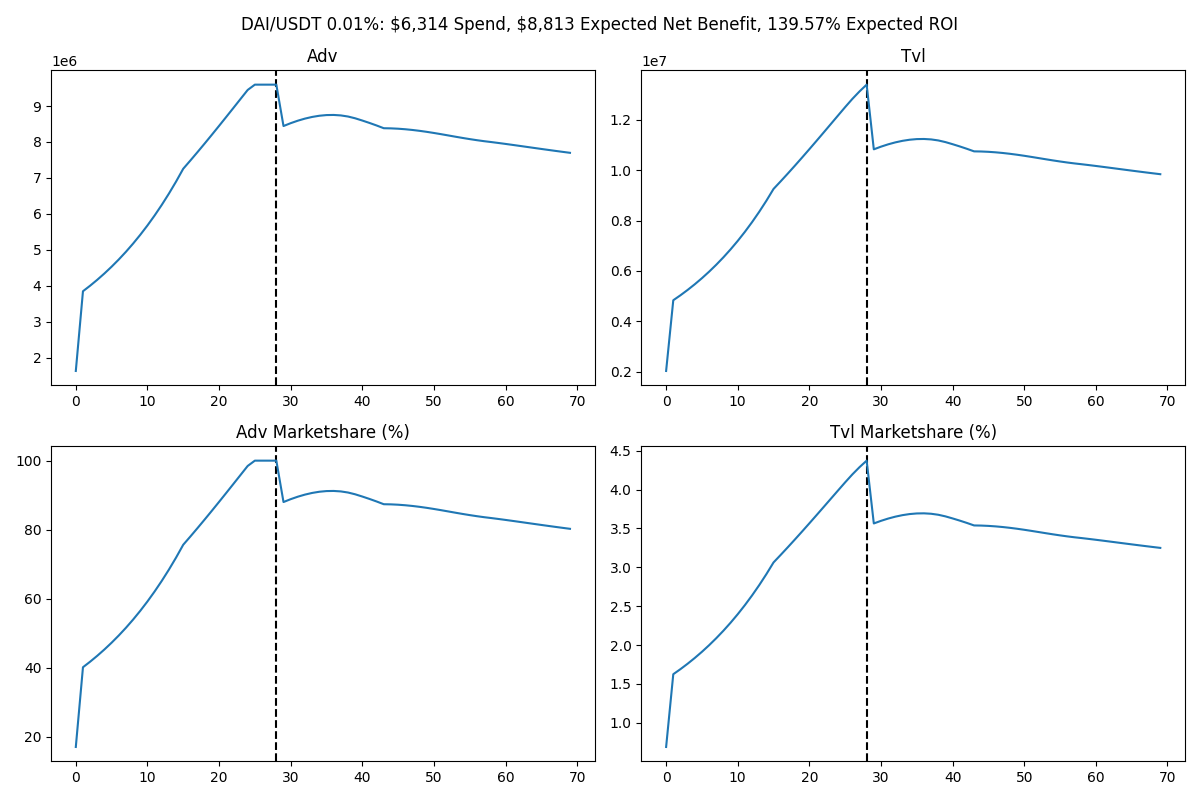
As expected, the introduction of incentives causes a big spike in TVL from LPs responding to the increase in net yield. This causes a proportional increase in ADV. During the experiment, there is a pinwheel effect of increasing ADV causing TVL to increase, and increasing TVL causing ADV to increase. Once the incentives end, TVL drops due to the decrease in net yield, which causes ADV to drop as well. But the equilibrium ADV and TVL is at a stable position well above where they were before the liquidity mining began.
The figure below demonstrates an unsuccessful liquidity mining scenario according to our simulation. Specifically, this simulation run corresponds to the METIS/WETH 0.3% pool given an incentive spend of $9170.
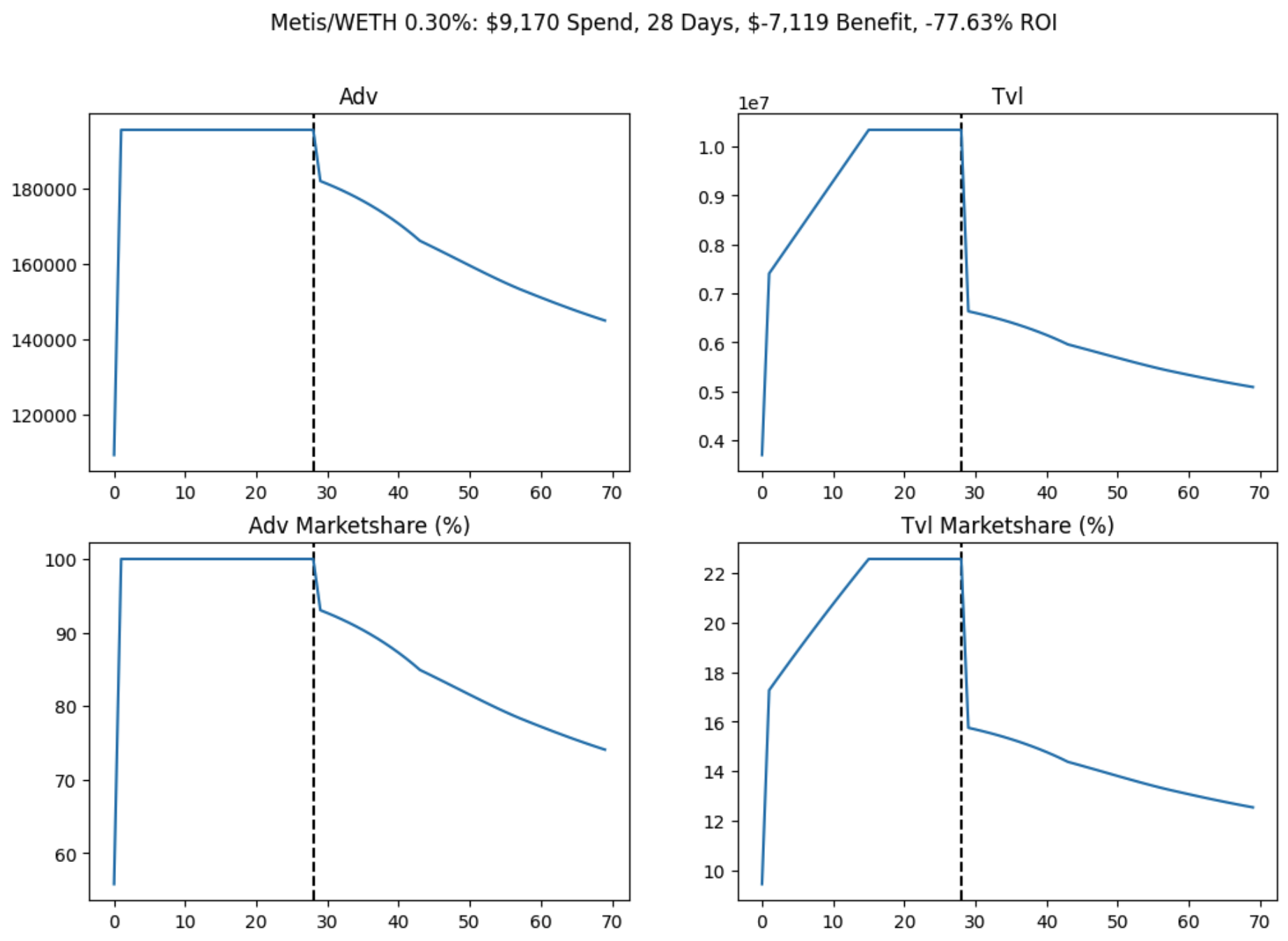
As before, the ADV and TVL increased in response to the incentives. However, due to the high ADV marketshare compared to the current TVL marketshare, the increase in trading volume was insufficient in incentivizing a high TVL in the longer term.
Parameter Selection
Choice of Pools: To determine which pools to incentivize, we started by identifying the trading pairs with the highest on chain 30 day trading volume. We then determined the top Uniswap V3 pool by volume for the top trading pairs, and computed its volume marketshare among competitors. We obtained a set of candidate pools by filtering this list down to the pools that compete with at least 1 non Uniswap pool and have a marketshare under 60%.
Incentive Spend: For each of these candidate pools, we optimized the incentive spend for the pool using our simulation. Specifically, we considered 100 multipliers against ADV from 0.01x to 100x on log scale, and used our simulation to estimate the net benefit. We dropped all candidate pools that were unable to provide positive benefit for any level of incentive spend. We used the best simulation result to determine an incentive spend for all pools we suggest moving forward with.
Incentive Period Duration: To determine the duration for running this incentive program, we look to the results of our Mainnet LP timing analysis, where we showed that the frequency at which whale LPs update their positions differs from pool to pool, ranging from once a day to once a month. In order to ensure that LPs have ample time to rebalance their positions during the incentive program, we conservatively recommend a duration of 4 weeks.
Example Pools
We are currently in the process of finalizing which pools to move forward with for the initial round of liquidity mining on mainnet, but we are able to share two examples of pools that look promising at this time: stETH/ETH 0.05% and SYN/ETH 0.3%.
Curve currently dominates the volume and liquidity marketshare of swaps between stETH and ETH. Preliminary data from our simulations suggests that with $153k of incentive spend, the protocol could receive a net $264k of benefit (assuming the fee switch is turned on), which represents an ROI of 173%.
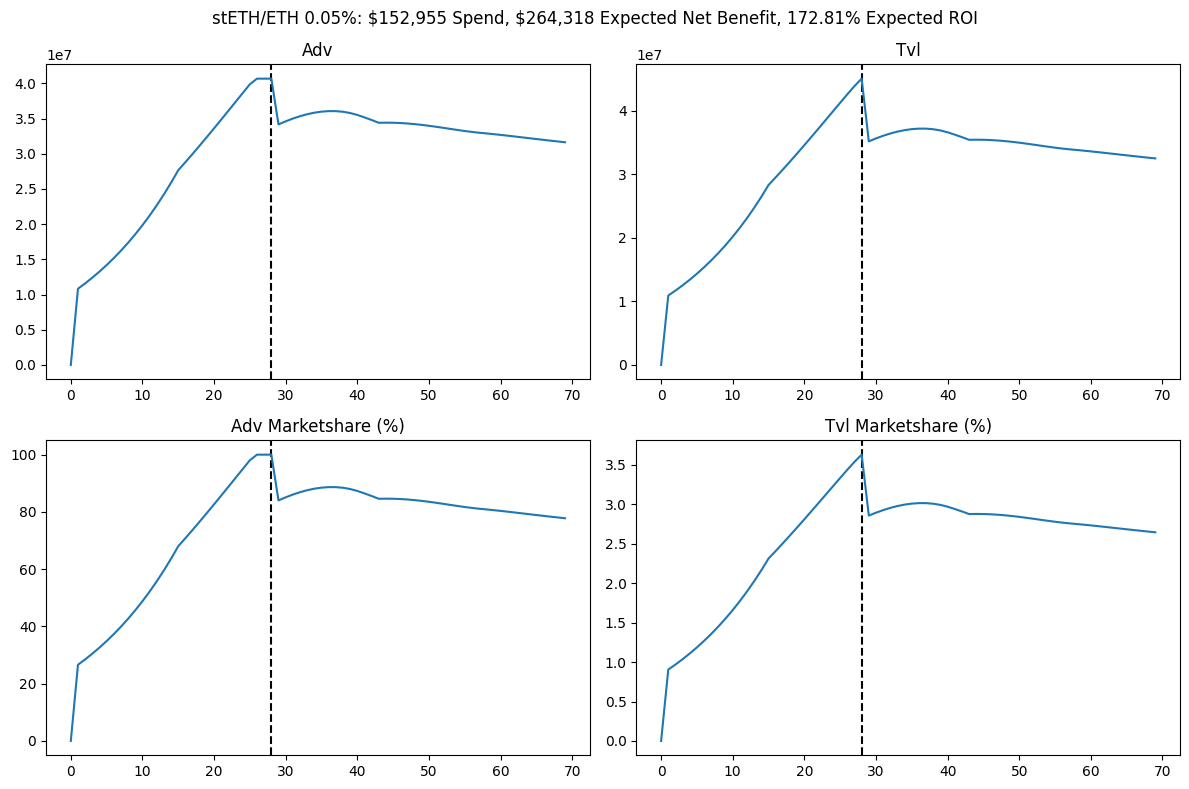
Sushi currently dominates the volume and liquidity marketshare of swaps between SYN and ETH. Preliminary data from our simulations suggests that with $31k of incentive spend, the protocol could receive a net $34k of benefit (assuming the fee switch is on), which represents an ROI of 114%.

Conclusion
Between the options of Trade Mining, Payment for Order Flow (PFOF), and Liquidity Mining (LM), our research shows that Liquidity Mining is the most promising approach to growing the Uniswap protocol. Our analysis showed that trade mining does not make sense because there is very little sticky trade volume on alternative DEXes that could be rerouted to Uniswap through a trade mining incentive program. A PFOF program could be helpful in incentivizing external exchange aggregators to integrate with Uniswap or indirectly incentivizing LPs to add liquidity by increasing returns, but it is less efficient than one time payments to external exchange aggregators or liquidity mining for LPs. Liquidity mining is effective because when executed correctly, LM incentives can bootstrap liquidity that sticks after incentives are eventually removed and drive long lasting volume. Uniswap’s most recent liquidity mining program that targeted pools on Optimism has had mixed results, but it lacked a scientific approach to picking pools which led to suboptimal results.
Gauntlet has established a methodology for identifying promising pools to incentivize and to predict the impact of LM on their volume and liquidity, which we will describe in a future post. The next steps for our Uniswap Foundation engagement are to finalize which pools to go live with and to work with a launch partner to set up the incentive program. We are planning on going live with this incentive program in the next few weeks.
Blog
View the full presentation
Read the full paper
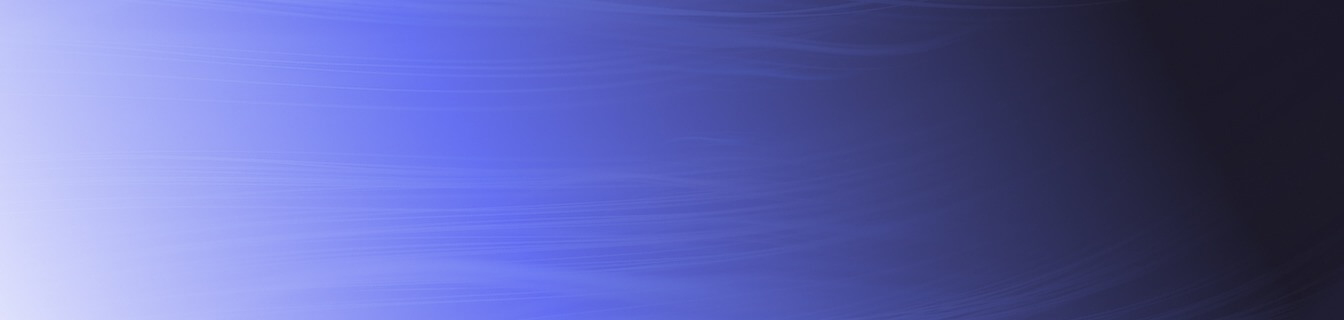