Executive Summary
Over a three-month period, Gauntlet executed a liquidity mining campaign on the Arbitrum network targeting Uniswap pools. Using 1M ARB tokens (approximately $1M USD at the time of commitment) and 100K UNI tokens (approximately $750K USD at the time of commitment), the campaign aimed to create a self-sustaining flywheel effect by attracting liquidity, improving price execution, and increasing volume market share on various Uniswap pools.
Overall, the campaign saw positive results during the program, which were sustained after the cessation of incentives.
During the Program:
- $14.85M in market share-adjusted daily TVL added to targeted pools
- $689 of TVL added per $1 of incentives
- $259M in market share-adjusted volume and $389K in LP fees added during the incentive program
Post-Program:
- $1.2M-$2.0M of daily TVL maintained in targeted pools post-incentives
- $214-$243 of TVL per $1 of incentives, including the program and post-program period
- Lasting volume growth for a majority (70%, 21/30) of the incentivized pools, which had a cumulative net gain of $735M in market conditions-adjusted annualized volume and a total of $1.6M in annualized liquidity provider (LP) fees across pools
Background
Program Details
The Arbitrum LTIPP (Long Term Incentive Pilot Program) is a three-month-long incentive program run by the Arbitrum DAO. Starting on June 17, 2024, a total of 45M ARB in incentives was distributed among grantee protocols with the goal of growing the Arbitrum ecosystem.
We collaborated with the Uniswap DAO-Arbitrum Delegate Program (UADP) committee to receive a 1M ARB grant through the LTIPP program that we used for a Uniswap V3 liquidity mining campaign. These incentives were matched by a 100K UNI grant from the Uniswap DAO, distributed alongside ARB incentives from July 15 onward. All incentives were distributed through Merkl by Angle Labs.
The Benefits of Liquidity Mining
The goal of this liquidity mining program was to grow the liquidity and volume of Uniswap pools on Arbitrum. In our prior Uniswap Incentive Design Analysis, we identified the Liquidity → Volume bootstrapping flywheel as the best way to generate lasting liquidity mining impact.

This works as follows:
- Liquidity mining incentives begin
- LPs add liquidity to the pool, which improves execution quality for traders
- Traders route more of their swaps through the pool and thus pay more fees to LPs
- Fees from traders attract further liquidity to the pool until a stable equilibrium between liquidity and LP returns (fees + incentives) is reached
- Liquidity mining incentives end
- Some liquidity is removed due to the reduced LP returns
- The final liquidity equilibrium is higher than it was pre-incentives because of greater fee incentives enabled by sustained trading volume
For this flywheel to work, the following must hold true:
- Liquidity must increase as a result of liquidity mining incentives
- Trading volume must increase as a result of deeper liquidity
Therefore, it is necessary to allocate incentives to pools for which LPs are elastic to incentive yield, and where traders are elastic to increases in liquidity. For this reason, we took a simulation-based approach to incentive allocation and projecting the lasting impact of incentives as opposed to simply spending incentives on blue-chip trading pairs.
Program Objectives
The goal of our previous Arbitrum liquidity mining program run for the Uniswap DAO was to increase the market share of Uniswap pools on Arbitrum. To do this, we built a methodology first to determine the necessary liquidity to best compete with other Arbitrum DEXs, and then optimize incentive spend to reroute the most volume.
For the LTIPP incentive program, with the Arbitrum DAO as the primary stakeholder, we designed a new optimization objective: maximize the ROI from our incentive spend on TVL and volume growth. Optimizing for TVL growth ensures that LPs are elastic to incentives and that incentives effectively deepen Arbitrum liquidity and bolster trading demand. This approach is aligned with driving the growth of the Arbitrum ecosystem.
Since it is crucial to ensure that this growth in volume and liquidity is sticky, we evaluated this growth a month after the campaign ended to assess what lift we can expect to retain in the longer term.
Methodology
Pool Selection and the Cold Start Heuristic
The first step in the process was selecting pools to include in the incentive program. We took a heuristic-based approach to identify potential targets, which focused on pools where Arbitrum was underrepresented in total trading volume for a given pair across competing chains. Our assumption was that this would make them strong candidates for growth.
Using data from the 30 days before June 10, one week before the start of the campaign on Uniswap, we aggregated the total volume by pair across various chains, including Arbitrum, Ethereum, Avalanche, BNB, Optimism, Polygon, and Base. To filter out low-activity pools and not overspend on pools with high existing market share, pairs were excluded if a) the volume on Arbitrum was less than $10,000, b) if the total volume across all other chains was below $10,000, or c) if the pair’s volume on Arbitrum exceeded 25% of the pair’s total volume across all chains. After calculating a ratio to illustrate each pair’s volume on Arbitrum against the pair’s total volume across all other chains, the 30 pairs with the lowest ratios were selected for incentive allocation.
Once pools were selected, the next step was to develop a distribution strategy for the initial tranche of incentives. We settled on distributing incentives proportionately based on the total volume of the pair on other chains while giving higher weight to pairs that appeared on multiple chains.
Model
Once we had the list of pools to be incentivized and their initial incentive allocation, we moved on to the second stage, which involved using a predictive model to reallocate incentives to pools with the greatest potential for TVL growth.
This model utilized data from past incentive performance to predict the impact of incentives in the future. The model does not simply calculate an unadjusted historic elasticity for each pool — it takes into account the diminishing returns from incentives and adjusts for the fact that larger, more stable pools tend to show lower TVL growth than smaller pools.
Optimal Incentive Allocation
During the campaign, we routinely reallocated incentives to ensure that the budget was spent efficiently on pools with the highest growth response.
The optimal allocation in each period was determined by solving a constrained optimization problem. We sought to maximize the amount of TVL across all incentivized pools while keeping the total incentive spend to the agreed-upon budget. The amount of TVL generated by a given distribution of incentives was predicted using the model described in the previous section.
To maintain a level of stability in the pools, the period-to-period changes in incentives provided to each pool were capped at 20% in either direction. This meant that pool allocations did not swing wildly from week to week and provided LPs with a certain level of predictability in terms of their expected returns from liquidity provision.
To standardize our distribution and responses to incentive provision in the form of two separate tokens — UNI and ARB — we measured the impact per dollar of incentive and did not distinguish between the tokens when calculating optimal allocations. As a result, the proportion of UNI tokens allocated to each pool is equal to the proportion of ARB tokens allocated to the same pools.
Performance Results
Total Value Locked (TVL)
During the Program
A comparison of the TVL before and during the campaign clearly shows that TVL grew considerably during the campaign. However, we need to examine how much of the increase in TVL was due to the campaign and how much of it was due to external market movements. To understand the impact of the incentive program, we establish an alternative baseline against which we can compare the actual observed growth in TVL. In other words, we want to answer the question — how much would TVL have increased in the absence of the incentive campaign over the same period of time?
We do not want to simply compare the TVL at the beginning, during, and at the end of the campaign. While this approach has the advantage of simplicity, it ignores the impact of market movements during the campaign period.
We can establish an alternative baseline by analyzing the set of all active pools on the Arbitrum network that did not receive incentives as part of the Uniswap LTIPP campaign.
The first filter used was to limit our interest to the pools that had updates to their LP positions on at least 200 days in the 2024 calendar year. There are 1,695 such pools that we refer to as continuously active pools and use as our baseline. Because there are significantly more continuously active pools than incentivized pools, the total TVL of the two groups will not be comparable. We can address this by normalizing the TVLs of both sets of pools so that they have the same average TVL from the beginning of the year to the start of the incentive period. We calculate the baseline TVL as follows:
\[TVL_{bl} = \frac{TVL_{ca}\times TVL_{inc}\left[2024-01-01, 2024-06-16 \right]}{TVL_{ca}\left[2024-01-01, 2024-06-16 \right]}\]
Here, \({bl}\) refers to the baseline, \({inc}\) to the incentivized pools, and \({ca}\) to the continuously active pools as defined in the previous paragraph.
We can then compare the value of this baseline TVL against the actual TVL of the incentivized pools over the incentive period. The difference between the two illustrates the impact of the incentive program on TVL. The chart below shows this comparison.
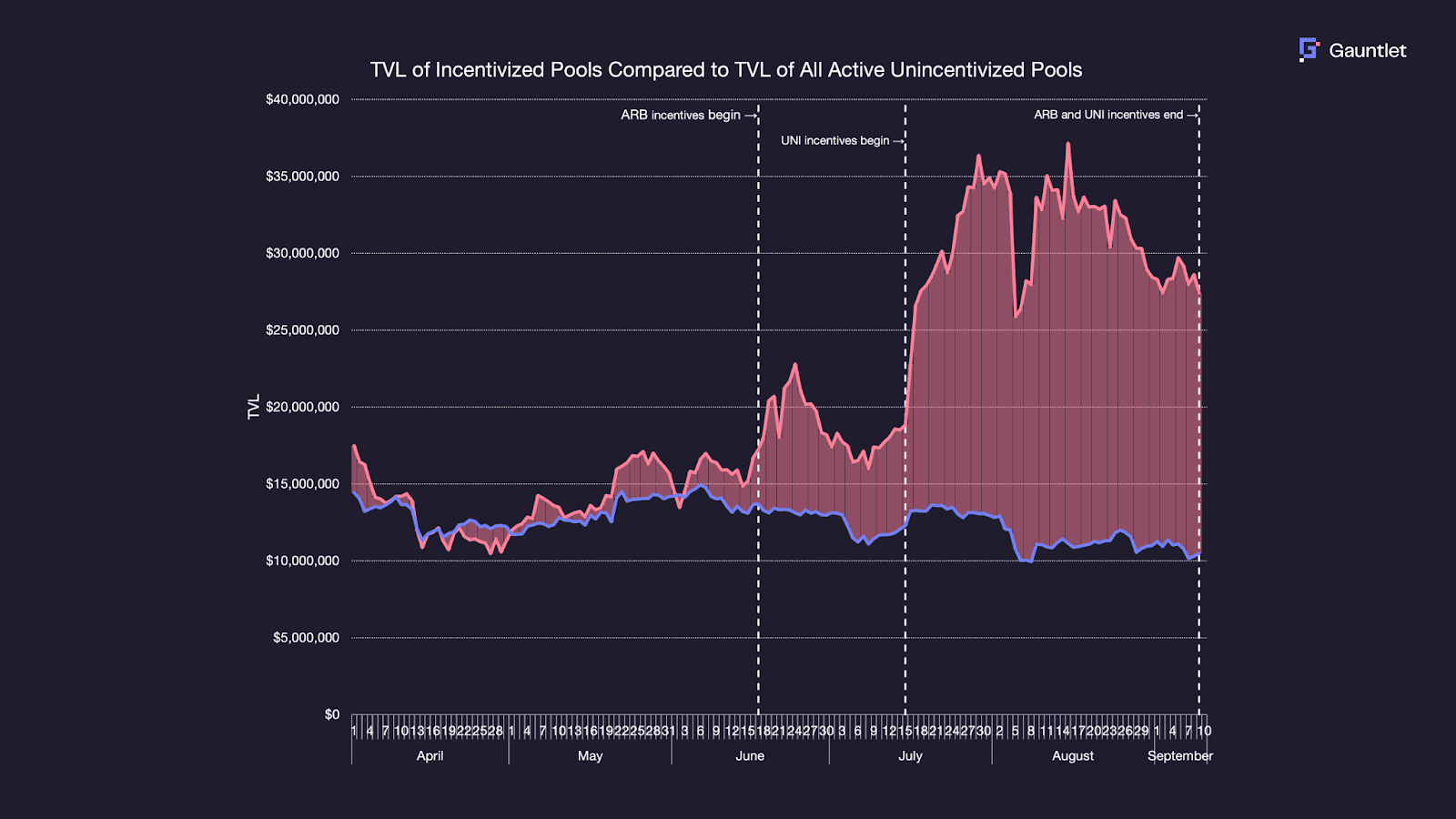
We can further improve our comparison analysis. The baseline includes many different pools that may be fundamentally different from the incentivized pools and may have had very different market forces acting upon them. In fact, some of these supposedly un-incentivized pools were part of different incentive campaigns during this period. For example, the gETH/WETH 0.5% pool, which is included in the baseline calculation, was part of a third-party incentive campaign that distributed 12,980 ARB tokens between March 22 and March 29, 2024.
Therefore, a better comparison approach would be to isolate the individual pools that most resemble the incentivized pools and use them to create a baseline. One way to do this would be to correlate the TVL between January 1, 2024, and June 16, 2024, of each of the incentivized pools against the TVLs of all un-incentivized pools, one at a time, and identify the pool that most closely matches the TVL movement of the given incentivized pool. We can further exclude any pools that have been a part of any previous incentive campaign on Merkl in the 2024 calendar year to ensure a clean baseline.
Once we have a comparable un-incentivized pool for each incentivized pool, we can normalize its TVL with the following formula:
\[TVL_{bl_i} = \frac{TVL_{uinc_i}\times \overline{TVL}_{inc_i}\left[2024-01-01, 2024-06-16 \right]}{\overline{TVL}_{uinc_i}\left[2024-01-01, 2024-06-16 \right]}\]
Here, \({bl_i}\) refers to the \({i}\) th baseline, \({inc_i}\) to the \({i}\) th incentivized pool, and \({uinc_i}\) to the \({i}\) th un-incentivized pool, which is the one most highly correlated with the \({i}\) th incentivized pool.
The sum of these normalized TVL series gives us the baseline to compare against the total actual TVL. The chart below shows how a set of TVL series constructed this way closely aligns with the movement of the actual TVL of the incentivized pools rather than the average TVL of all un-incentivized pools, as we had calculated in the previous step.
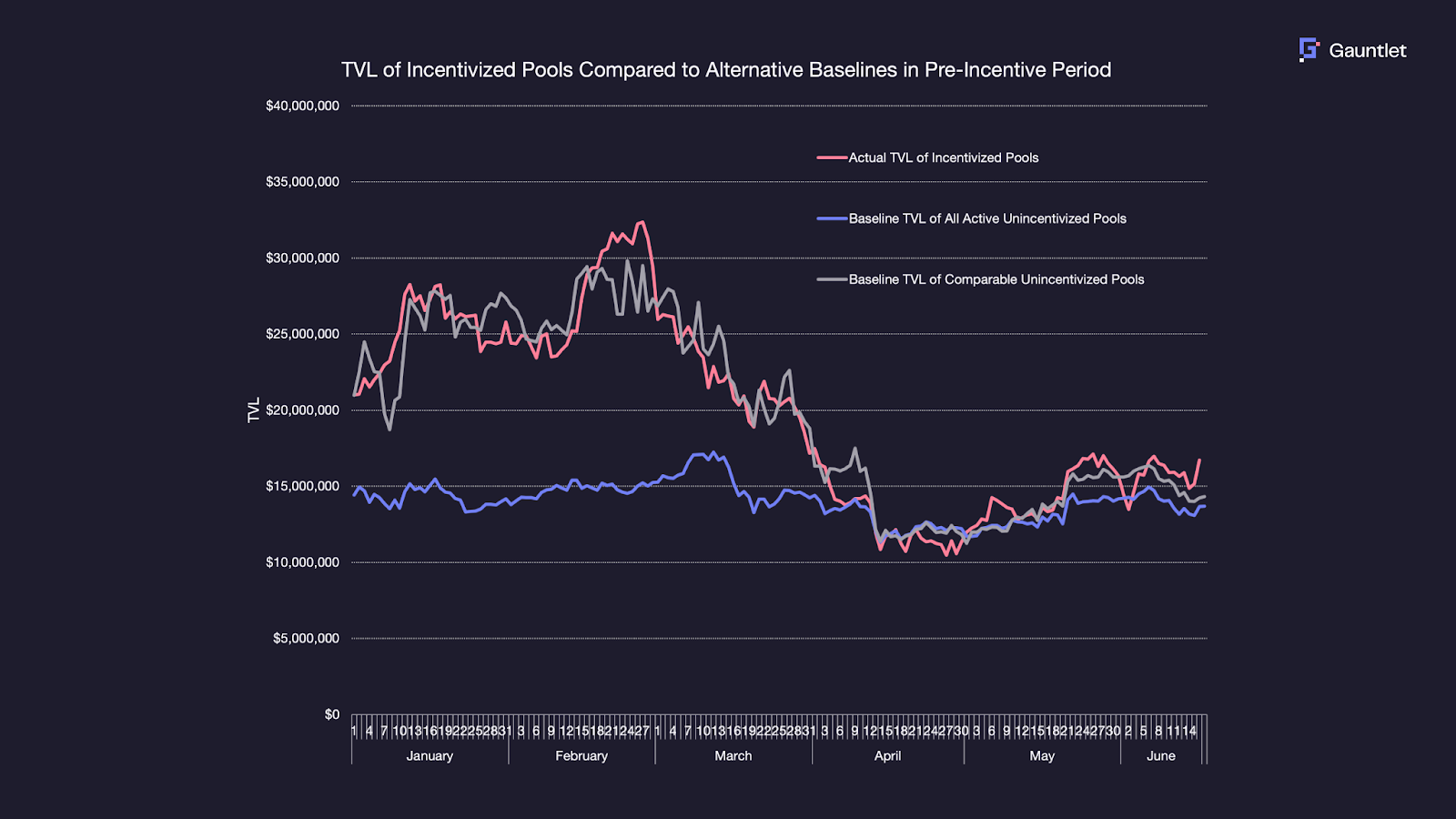
We can then compare the actual TVL of the 30 incentivized pools against the total normalized TVL of the 30 comparable un-incentivized pools, and the difference should give us an idea of the impact of the incentive program.
The chart below shows the two series, and the shaded pink area represents the increase in TVL observed in the incentivized pools, which can be attributed to the incentive program. According to these calculations, the total TVL of incentivized pools was $15M higher per day on average over the campaign period than it would have been without the incentive program.
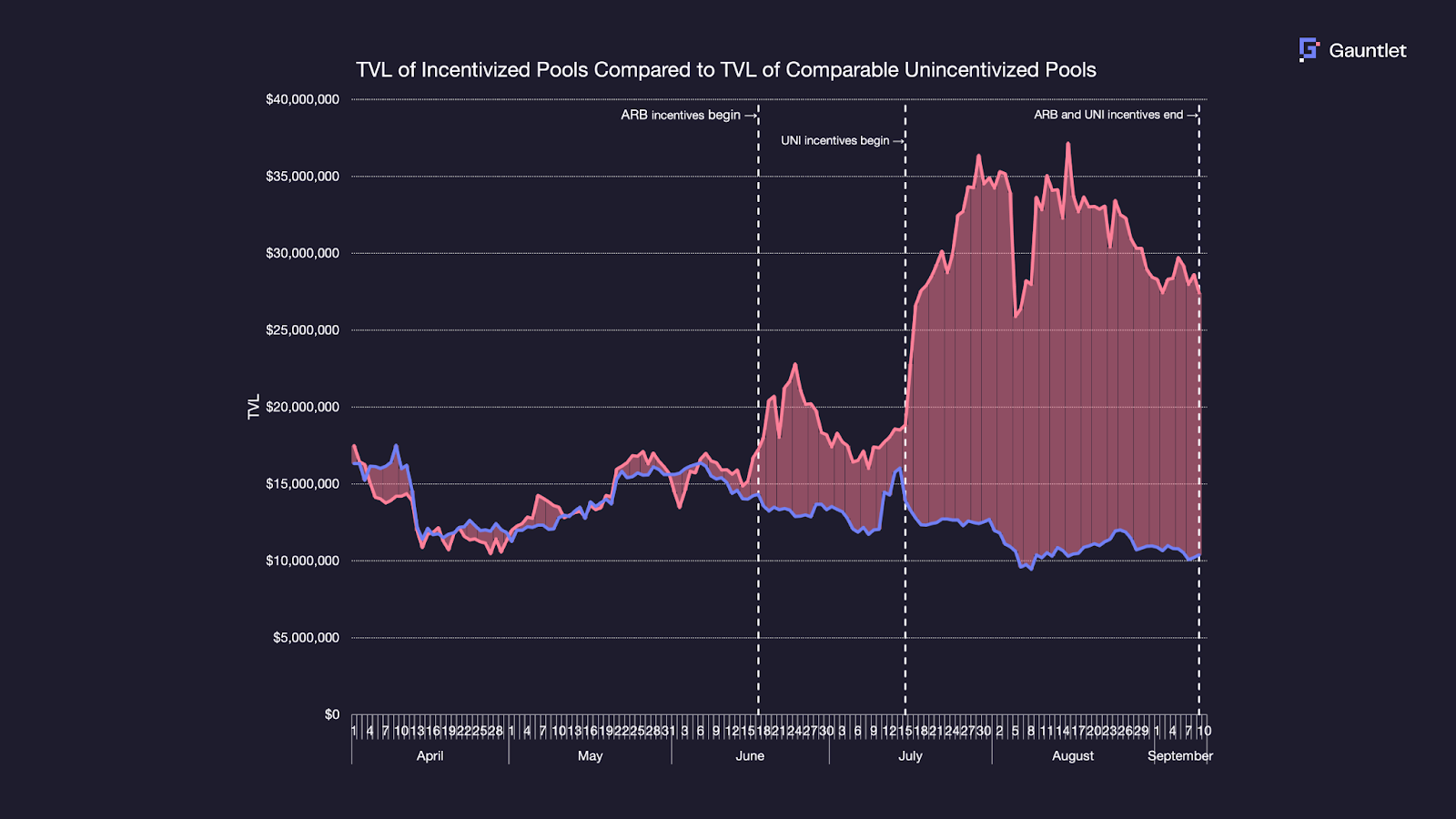
While we cannot cleanly isolate the impacts of the ARB and UNI incentives separately, we observe that during the period when only ARB incentives were in effect, the TVL of incentivized pools was $5.4M higher per day on average than it would have been. When ARB and UNI incentives were both in effect, the TVL of incentivized pools was $19.6M higher per day on average than it would have been. Therefore, the introduction of UNI incentives effectively provided even more substantial gains in TVL.
After the Program
While we cannot predict the continued impact of the incentives after the program ends, we can use the information available to us to come up with a defensible estimate. Typically, when an incentive program ends, we see a drop-off in TVL and, consequently, in volume. In the past, we have observed that the decline in incremental TVL tends to follow a power law distribution, i.e., starting with a sudden large drop and then declining at a slower rate, asymptotically approaching an equilibrium.
Examining the three weeks since the end of this program, we see that this pattern holds. The chart below shows the difference between incremental TVL (i.e., the difference between the actual TVL and the baseline TVL), represented as a percentage of the baseline TVL. The pink curve shows the power distribution that best fits these data points.

According to this model, we expect the incremental TVL to fall from above 150% of baseline TVL to around 50% of baseline TVL in about a week and then continue to fall at a much slower and decreasing rate. By June 2025, we expect incremental TVL to be around 3.5% of baseline TVL. If we consider the period from September 30, 2024, through June 16, 2025 (i.e., one year from the start of the incentive program), we can expect incremental TVL to be at an average of 13% above baseline TVL over the entire period.
To estimate the average incremental TVL one year after the end of the incentive program, we need an estimate for the growth in baseline TVL over the same period. The simplest way to do this is to assume that baseline TVL will continue to grow after the end of the incentive program at the same rate that it grew before the program. For this purpose, we use the median of the day-on-day growth rate in TVL between June 17, 2023, and June 16, 2024 (the day before the start of the campaign). This comes to a rate of increase of approximately 0.326% per day, as can be seen in the chart below.
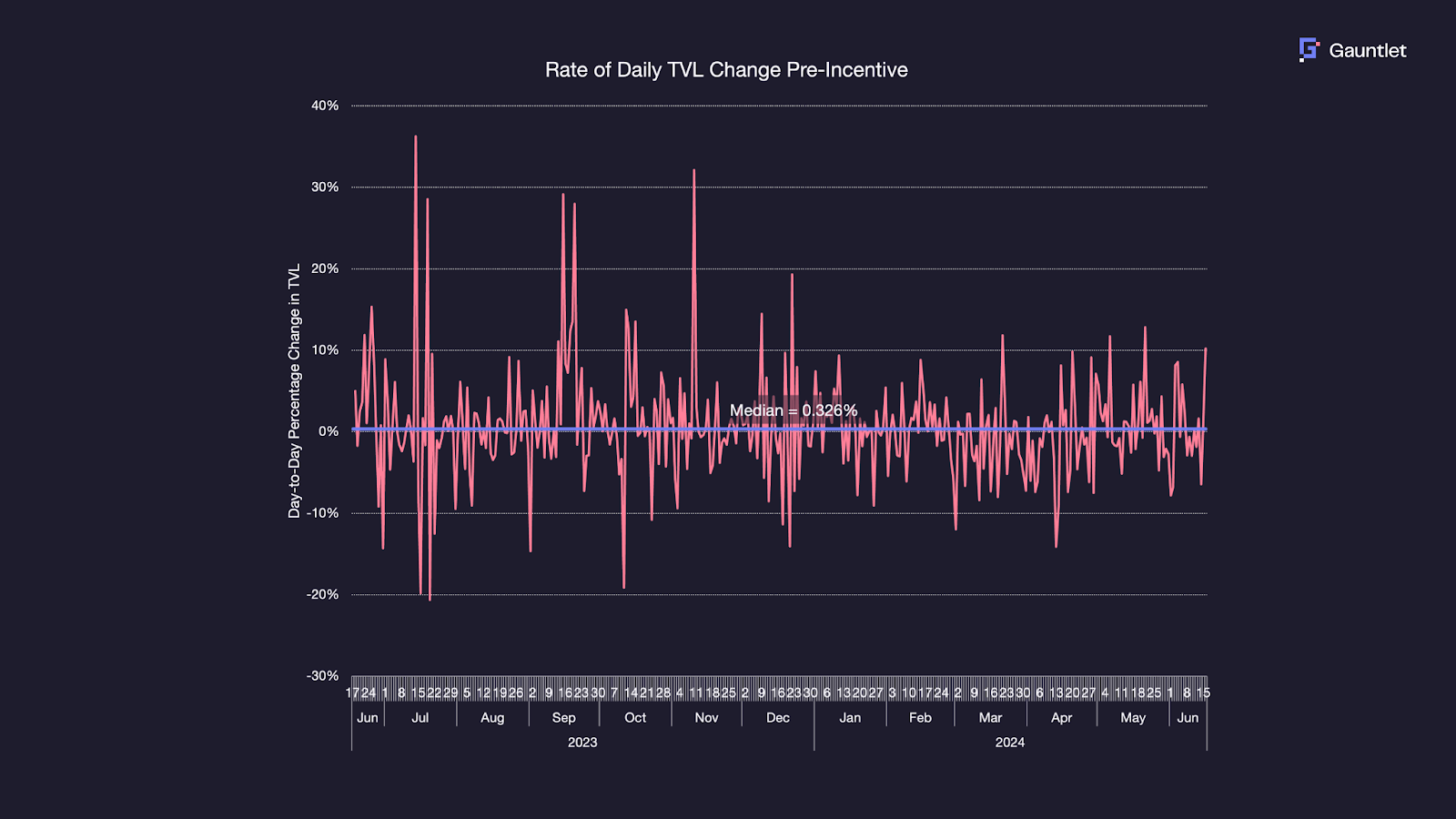
Putting it all together, the following chart shows the incremental TVL that would persist in the incentivized pools over the year following the incentive program (assuming that baseline TVL grows at the same rate as it did before the incentive program). The pink shaded area between the estimated TVL with incentives and the estimated baseline TVL lines represents the estimate of the incremental TVL.
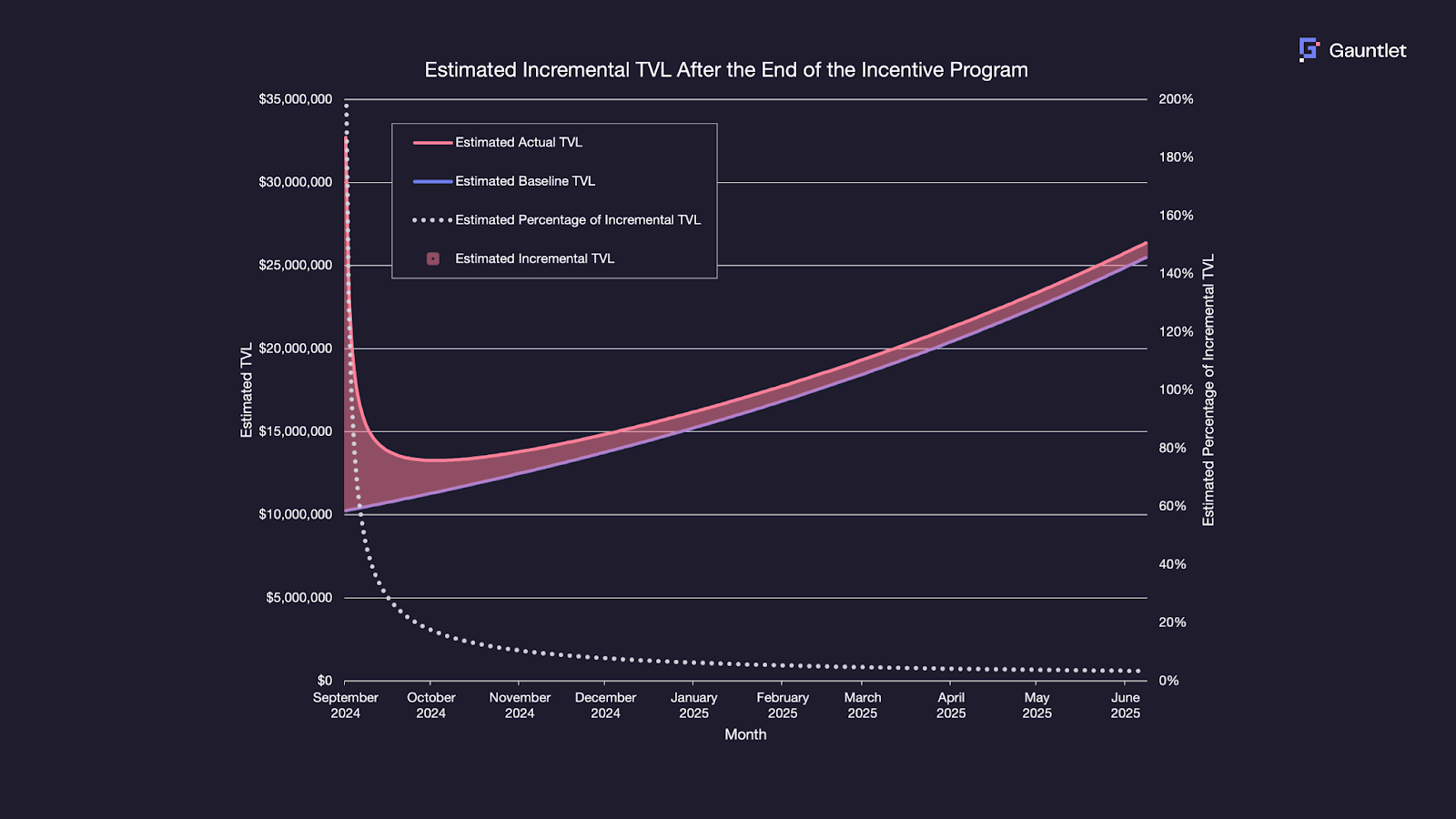
Under this assumption of TVL growth, we estimate an average daily incremental TVL of approximately $1.4M for the year after the program ends. This incremental TVL is unevenly distributed, starting from a peak and slowly declining to around $875K by the end of the year.
However, this is not the only possible outcome. The total amount of incremental TVL will change depending on the actual growth of baseline TVL over the next year. In the chart below, we show the amount of incremental TVL under three different assumptions of baseline TVL growth — the “moderate” growth assumption, which is the median value of day-on-day growth that we used in the previous exercise; the “low” growth assumption, which is half the value of the median growth rate (i.e., 0.163%); and the “high” growth assumption, which is twice the value of the median growth rate (i.e., 0.652%).
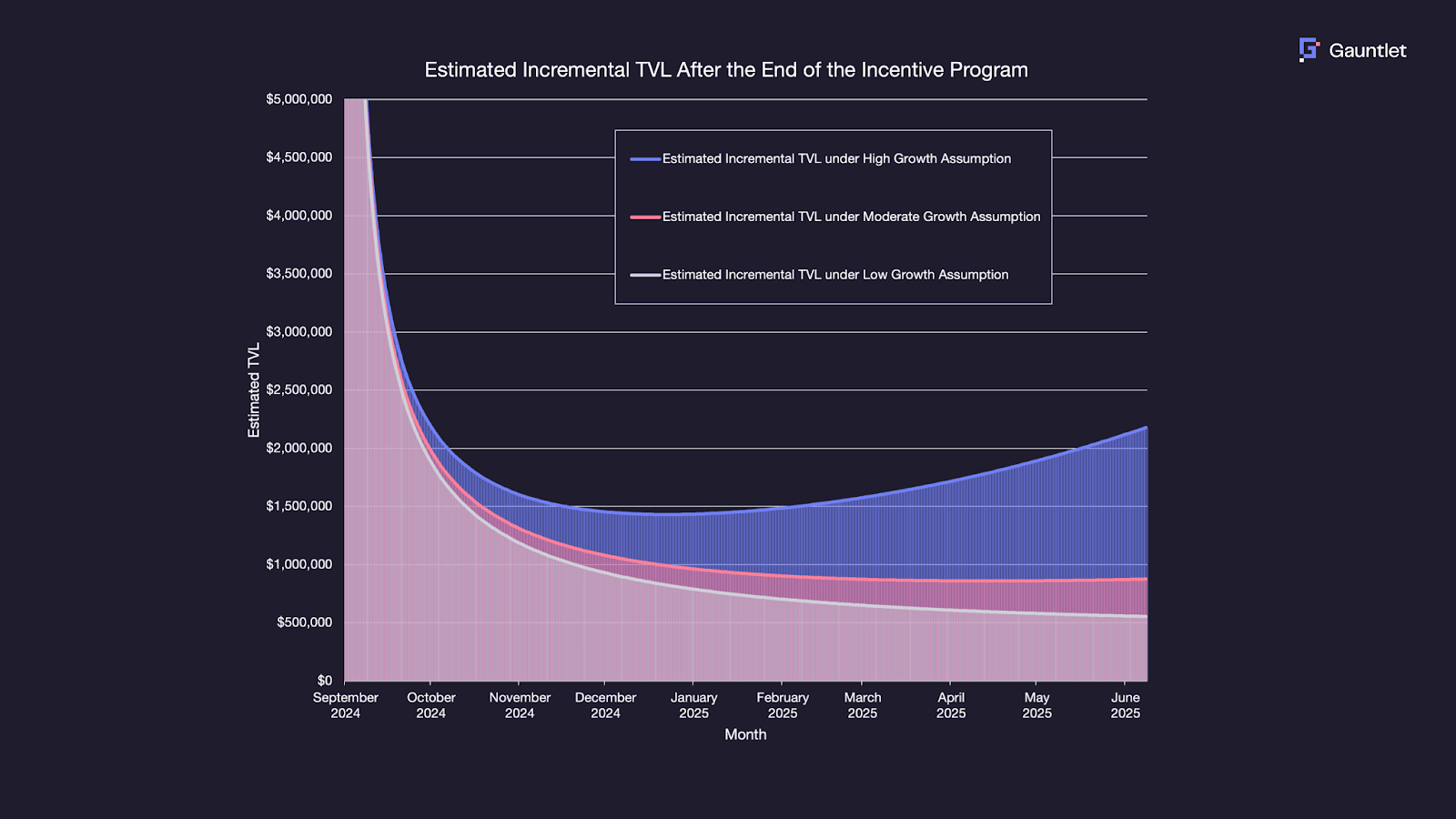
As seen in the charts above, if TVL grows organically at a high rate, the impact of the incentive program could remain high up to a year after it ends.
Under the high growth assumption, the incremental TVL would be $2M, while under the low growth assumption, it would be $1.2M.
Instead of using twice and half the median growth rate as the high and low growth rates, we examined the possibility of using the 75th and 25th percentile day-on-day growth rates instead. However, this approach led to an unreasonably large rate of growth under the “high” assumption (2.9% per day) and an unreasonably low rate of growth under the “low” assumption (-2.3% per day), so we did not pursue it.
Summary

Volume
The figure below shows the daily trading volume for each of the 30 pools from one month before the incentive period to one month after. The dashed lines show the start and end of the incentive campaign.

Most pools experienced a lift in volume during the incentives period compared to pre-incentives, but some pools retained stickier volume after the incentives period than others. The table below quantifies the change in average daily volume (ADV) experienced by each pool during and after the incentive period relative to before the incentive period.

Looking at the aggregate Uniswap V3 Arbitrum volume data for non-incentivized pools, there was a drop in average daily trading volume (ADV) of 10.57% between the incentive period and pre-incentive period and a drop of 33.65% between the post-incentive period and pre-incentive period. Therefore, to normalize the pool-wise measurements of ADV, we can multiply our measurements of ADV during the incentive period by the ratio of market volume before and during the program. We then multiply our measurements of ADV after the incentive period by the ratio of market volume before and after the program. The table below uses this adjustment to compute the change in volume for these pools.
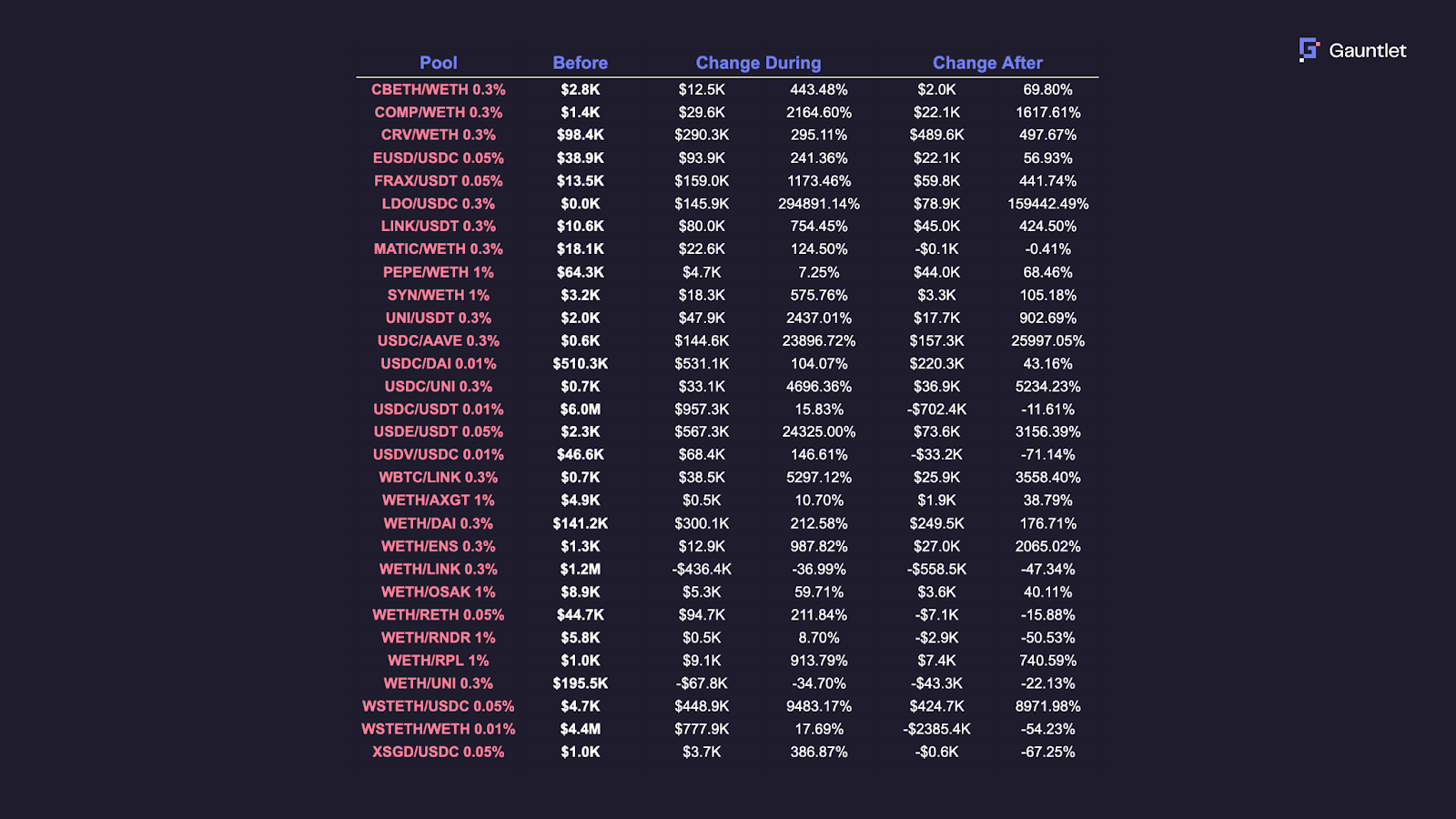
Based on these adjusted numbers, all pools but two (WETH/LINK 0.3% and WETH/UNI 0.3%) experienced a boost in volume during the incentive program. Several pools received particularly large percentage increases in volume, mainly inactive pools with negligible volume that were brought back to life (LDO/USDC 0.3%, USDC/AAVE 0.3%, USDC/UNI 0.3%).
After the incentive program, 21/30 pools ended up with a positive change in adjusted volume, which indicates that the program succeeded in driving lasting volume for most of the incentivized pools. Compared to the baseline volume from before the incentive program, these 21 pools collectively contributed a total gain of $259M in volume (and $389k in LP fees) aggregated across the days of the incentive period. This contributed to an annualized gain of $735M in volume (and $1.60M in LP fees) after the program ended using the post-incentive volume numbers.
Active Management
Model Accuracy
Our model predicted the TVL of the 30 incentivized pools with a high degree of accuracy. The chart below shows the actual weekly average TVL for each week that the program was in effect and the average weekly TVL predicted by the model for the given week based on the data as of the prior week. As can be seen from the chart, the model’s accuracy increased as more data became available.

Rebalancing
We dynamically optimized the pools to which LTIPP incentives were distributed — the share of incentives allocated to each pool changed with every new tranche of incentives. We used the prediction model to estimate the effect of a dollar of incentives on each pool. Based on those estimates, we calculated an optimum allocation of incentives across all 30 incentivized pools. This allowed us to react to information provided by LPs in the form of their responsiveness to incentives and change course as necessary.
As an example, if we had not rebalanced the pools every two weeks and instead let the initial allocation remain in place for the duration of the campaign, the TVL in the pools would have been lower on average by $1.8M over the duration of the campaign. The chart below shows the predicted TVL if the initial allocation had been maintained for the entire campaign and compares it to the predicted TVL based on the actual incentive allocation.
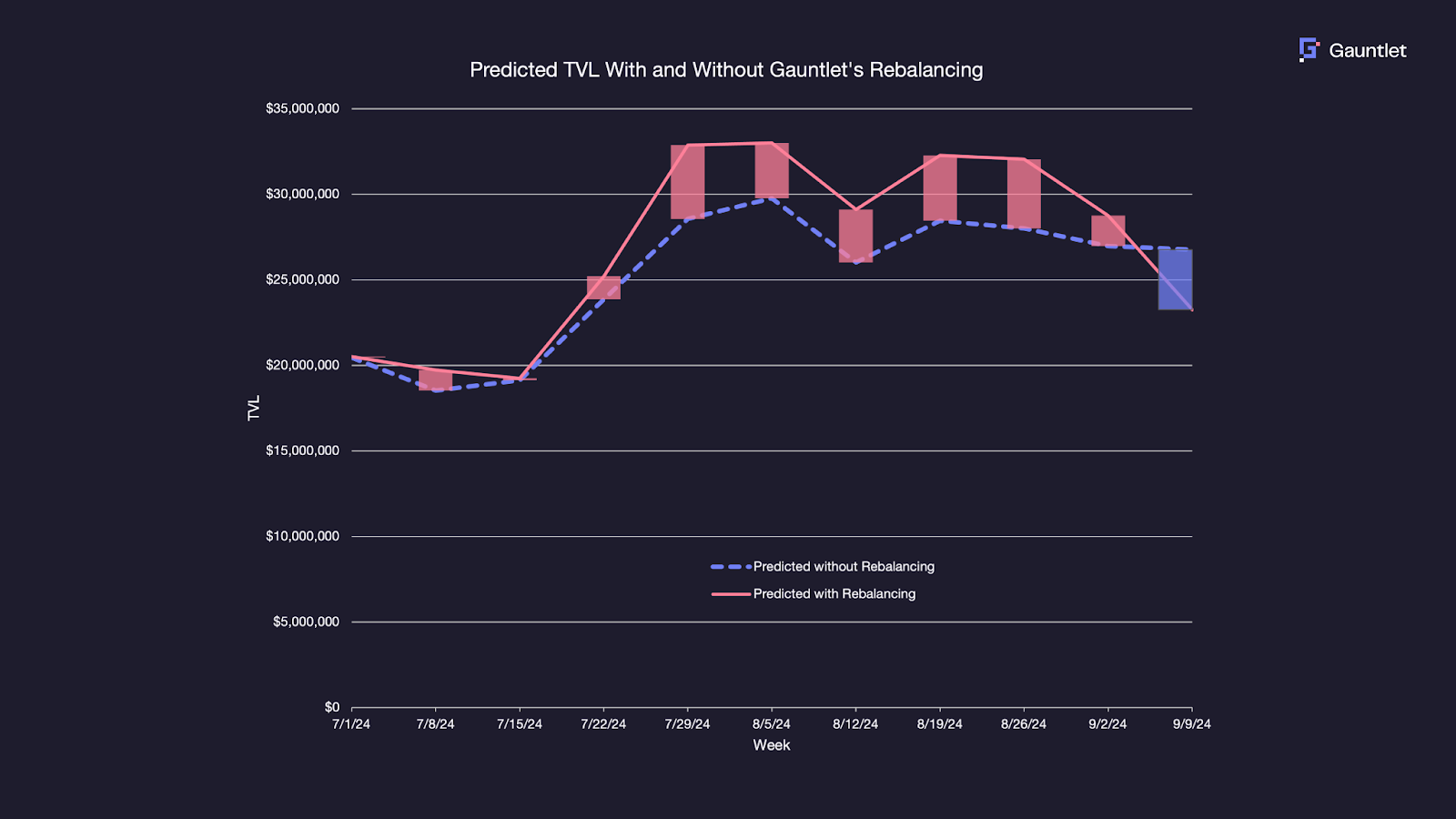
Insights, Observations, and Best Practices
Rebalancing Strategy
The overall recommendation from our model was to focus on supporting mid-size, growing pools with more incentives.
Larger pools, like USDC/USDT and WETH/wstETH, showed high elasticity in response to incentives but were eventually given fewer incentives due to their already significant TVLs. During market corrections, larger pools experienced sharp drops but showed rapid recovery, suggesting high elasticity. The large pools also maintained their TVL in some instances, even after incentives were reduced.
Smaller pools, such as USDC/XSGD and MATIC/WETH, showed greater percentage increases in TVL when given more incentives (147x and 10x, respectively, by the end of the incentive period), prompting a reallocation toward them. Pools with low TVL also benefited more from incentives than larger pools.
The incentives were gradually reallocated from larger, more established pools to mid-sized pools showing growth potential based on the observed impact of incentives. Smaller pools, especially those receiving more incentives, showed significant TVL growth, highlighting the effectiveness of the incentive reallocation strategy, while large pools continued to sustain themselves with fewer incentives.
Changes Due to UNI Incentive Boost
As mentioned in previous sections, the incentives provided were initially in the form of ARB tokens. After these ARB incentives had been in place for four weeks, an additional UNI incentive grant was made available. For the following eight weeks, the ARB and UNI incentives existed side by side.
While the ARB incentives had already boosted the TVL on incentivized pools, the addition of UNI incentives significantly impacted TVL beyond that. While this is expected since the addition of the UNI tokens considerably boosted the total dollar amount of incentives, what is notable is that the increase in TVL per dollar of incentives provided was also higher during the period of ARB and UNI incentives ($694) than it was during the period of only ARB incentives ($656). We cannot accurately quantify the relative value of UNI vis-a-vis ARB because there was no period during which only UNI incentives were offered. Still, the difference in value appears significant, suggesting that the value to LPs of a dollar’s worth of UNI is higher than that of a dollar’s worth of ARB.
We did not treat the two types of incentive tokens any differently, choosing instead to focus on their dollar value. In a future incentive campaign, we may incorporate the differential impact of tokens into our model.
Pool Observations
When we measured the net change in market-adjusted volume gain post incentives, the highest-performing pools in this program were CRV/WETH 0.3% (+$490K), wstETH/USDC 0.05% (+$425K), and WETH/DAI 0.3% (+$250K). The worst performing pools were WETH/LINK 0.3%, USDC/USDT 0.01%, and wstETH/ETH 0.01%, which all lost volume post incentives.
We found in our previous Arbitrum Uniswap liquidity mining program that LM incentives are effective in providing lasting volume growth to pools with little usage. Our results confirm this observation, with the four pools that started with under $1K in average daily volume (WBTC/LINK 0.3%, USDC/UNI 0.3%, USDC/AAVE 0.3%, LDO/USDC 0.3%) each experiencing lifts in volume even after incentives were removed. Collectively, these pools grew their market-adjusted average daily volume (ADV) from $2K to nearly $300K when comparing the pre-incentive period to the post-incentive period.
In the past, we also found that larger cap pools with blue chip assets tend to have a higher elasticity to incentives than pools for lower cap tokens, which is even more pronounced for stable pairs. This tendency can also be logically derived from the fact that lower cap tokens tend to be more volatile, leading to more LVR and impermanent loss for LPs, so the LPs for lower cap tokens would require a higher yield to compensate.
Interestingly, while incentives are highly effective in causing a short-term boost in pool usage during the incentive period for these high-cap stablecoin pools, these pools had some mixed results regarding stickiness after incentives. The USDC/USDT 0.01%, wstETH/WETH 0.01%, and USDV/USDC 0.01% all experienced drops in market-adjusted ADV after incentives were removed to below pre-incentive levels. However, the USDe/USDT 0.05%, USDC/DAI 0.01%, and FRAX/USDT 0.05% pools experienced significant gains that continued after the incentive period.
Simply incentivizing these larger pools may not always be the best approach for driving lasting growth. We hypothesize that the often mercenary nature of stable pair LPs may come from the highly competitive yield farming landscape, causing LPs to be highly elastic to changes in yield.
Importance of Goal-Driven Incentives
The success of this program highlights the effectiveness of a goal-oriented approach in driving sustainable growth. By setting clear objectives around liquidity growth and market share, we were able to strategically allocate incentives, resulting in stronger retention of activity and liquidity even after the program ended.
This outcome underscores the value of designing incentive programs with specific goals, as it encourages participants to engage in ways that contribute to lasting ecosystem benefits. We advocate for similar approaches in future programs to maximize stickiness and ensure that each incentive dollar fosters enduring impact.
Conclusion
Summary
The LTIPP program was conducted by the Arbitrum DAO in collaboration with the Uniswap DAO. The aim was to stimulate growth in the Uniswap ecosystem by attracting liquidity, improving price execution, and increasing trading volume on various Uniswap pools.
The program allocated 45M ARB in incentives to grantee protocols. In partnership with the Uniswap DAO, Gauntlet received 1M ARB for a liquidity mining campaign. Incentives were distributed to targeted Uniswap V3 liquidity pools via Angle Labs.
The campaign started on June 17, 2024, and over the next 12 weeks, we distributed incentives based on a predictive model that allocated more incentives to pools with a greater potential for TVL growth. The pools were initially chosen using a heuristic approach based on their potential to capture market share.
The program actively rebalanced the distribution of incentives based on pool performance and responsiveness to incentives. As the program progressed, larger pools received fewer incentives, while mid-sized and smaller pools received more incentives to maximize impact.
Despite market fluctuations, the program significantly increased TVL and trading activity. TVL increased significantly during the campaign, with incentivized pools experiencing $15M higher daily TVL compared to baseline estimates, translating to $690 in TVL per dollar spent on incentives. After the program ended, TVL declined sharply, but going forward, a gradual decline in incremental TVL is expected, so that TVL will remain above baseline levels for up to a year.
Volume growth closely correlated with TVL growth. For every 1% increase in TVL, a 0.92% increase in trading volume was observed. The program’s impact on trading volumes also translated into higher fee generation for Uniswap.
What’s Next?
With the LTIPP program now concluded and all incentives fully distributed, we are prepared to build on the insights gained from this initiative to inform future incentive programs. Leveraging the data and outcomes observed here, we will refine our approach to designing and executing effective incentive strategies that drive sustainable growth.
We look forward to continuing our collaboration with Uniswap, Arbitrum and the larger DeFi community to develop robust, data-driven programs and to share ongoing insights that will help optimize the impact of incentives across ecosystems. We extend our gratitude to the program operators and the DAO for their partnership and trust in this endeavor.
Blog
View the full presentation
Read the full paper
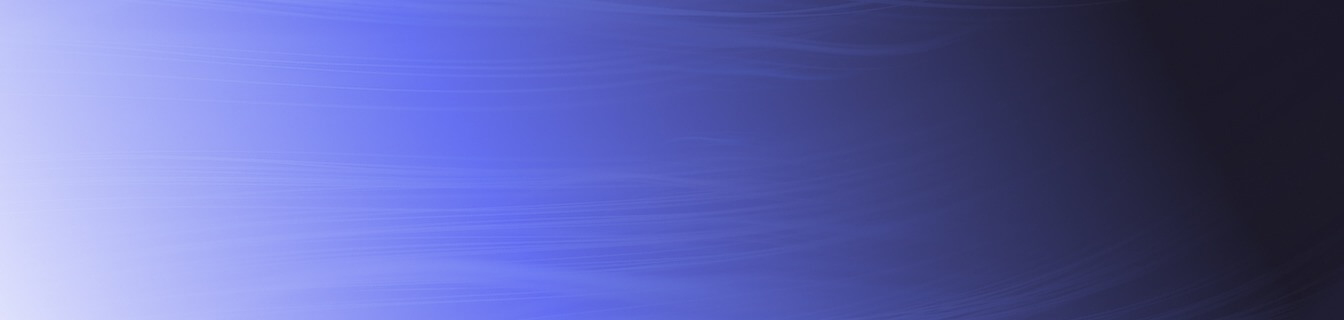